IoT Predictive Maintenance
Find, fix, and foster effective operations with IoT Predictive Maintenance Services
By considering asset reliability, Opsio’s experience on IoT for predictive maintenance uses intelligent data insights to monitor and maintain operations with early fault detection and minimal downtime.
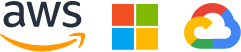
Introduction
Ensure operational longevity with intelligent IoT predictive maintenance by Opsio
As the market landscape becomes more competitive, enterprises begin to manage assets in smart ways and with tools. However, businesses still rely on traditional maintenance methods, which often lead to costly downtimes or unnecessary repairs.
To combat these hiccups, IoT predictive maintenance is instrumental in delivering real-time machine intelligence that stops problems in their tracks. Our expertise and solutions in this advanced approach help companies decrease their operational downtime and extend asset life while improving efficiency over time.
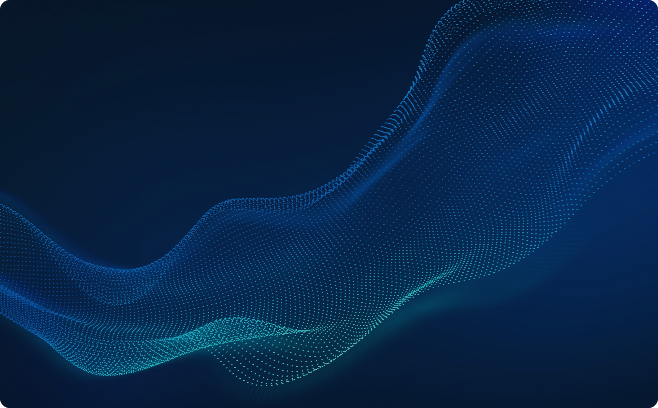
24/7/365 days connected assets,
continuous Performance
What is IOT predictive maintenance?
Swiftly achieve operational intelligence with IoT predictive maintenance services
IOT predictive maintenance functions as a black box of operations, not just to record disrupting events but also to stay ahead of them. It uses smart sensors and cloud-connected systems to connect data from multiple tech devices, platforms, software, and systems connected via one another to monitor equipment in real time. Instead of relying on fixed timelines for repairs, this advanced maintenance strategy helps industries reduce unnecessary costs and increase uptime.
Why do ongoing businesses need IoT predictive maintenance?
Achieve your business growth with reliability with Opsio's IoT predictive maintenance services
There is no doubt that every business struggles with major challenges, combined with unexpected breakdowns and rising maintenance costs. Having data alone isn’t enough; businesses need intelligent systems that act in real time. Opsio takes it one step further by utilizing a rule-based framework, combining analytics, AI and ML algorithms to predict issues and store data in the cloud environment. These approaches help provide actionable insights, foster decision-making, and reduce maintenance costs.
Our Services
Predictive maintenance solutions tailored for your industry
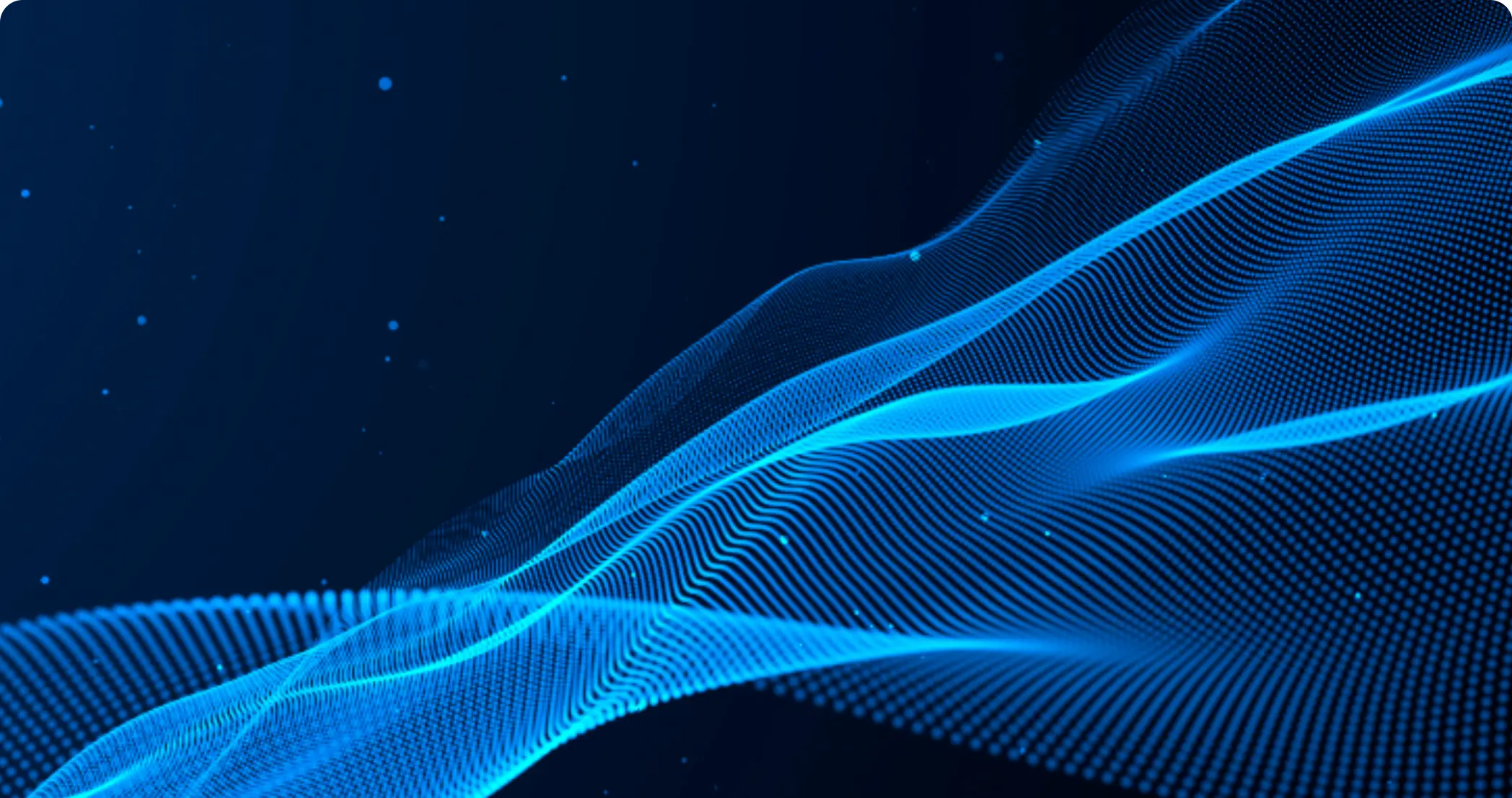
Automate your workflow maintenance
Manually finding the repairs can often result in project delay and miscommunication. Our expert team provides IoT solutions that enable your operations to connect their sensors, smart machines, and insights to existing ERP or CMMS systems for workflow maintenance and optimization.
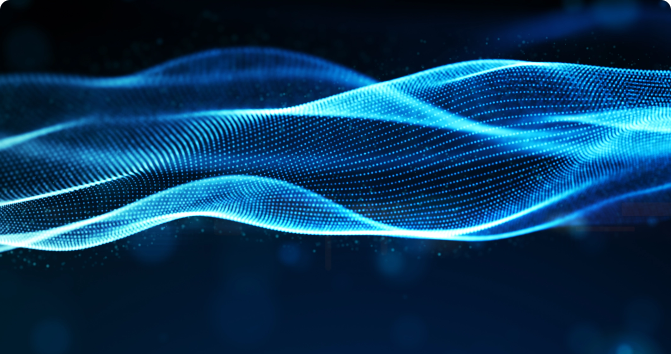
Reduce Maintenance Costs
Save on costs by addressing issues before they escalate.
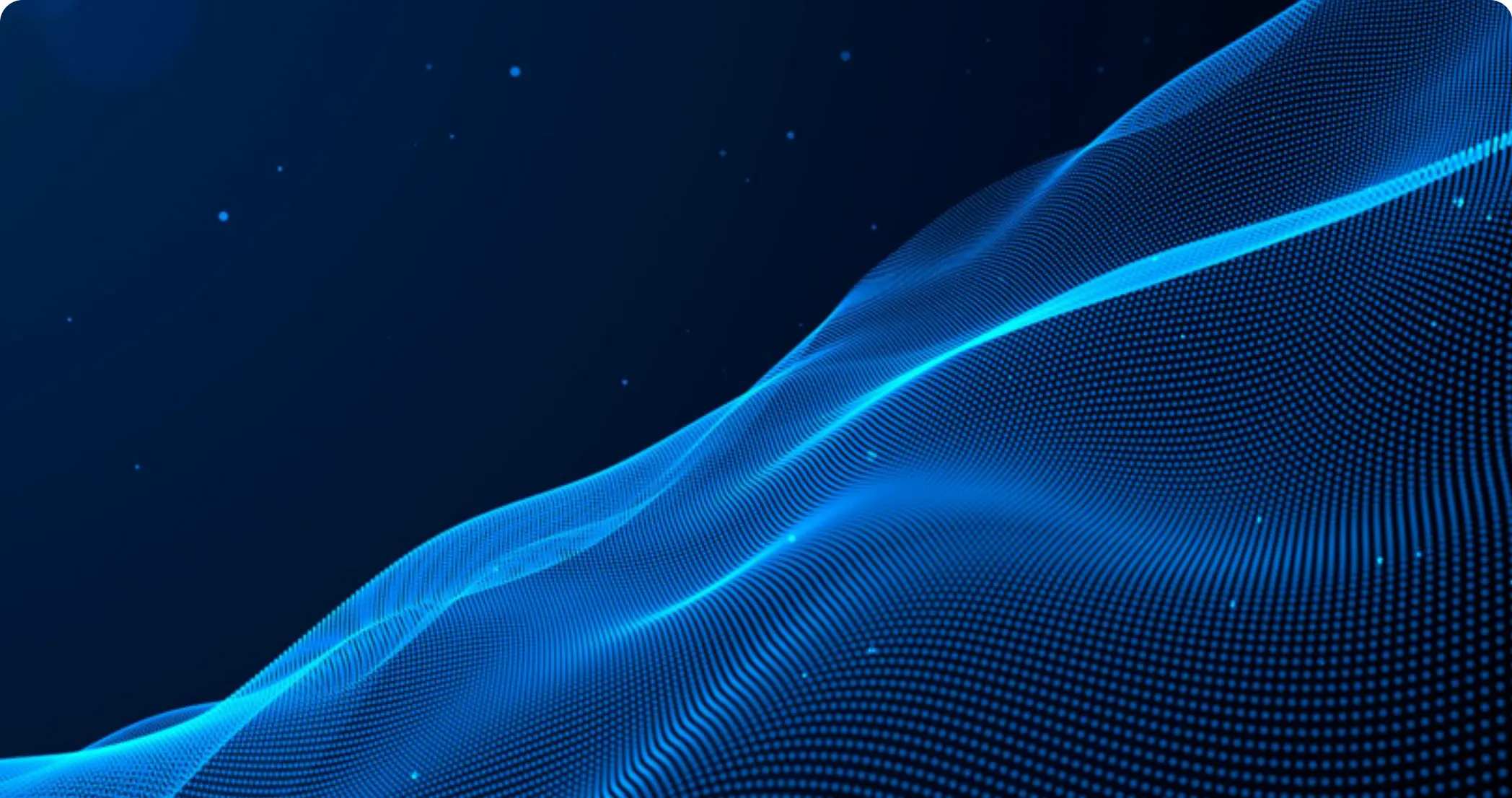
Extend Equipment Lifespan
Prolong the life of your machinery with timely interventions.
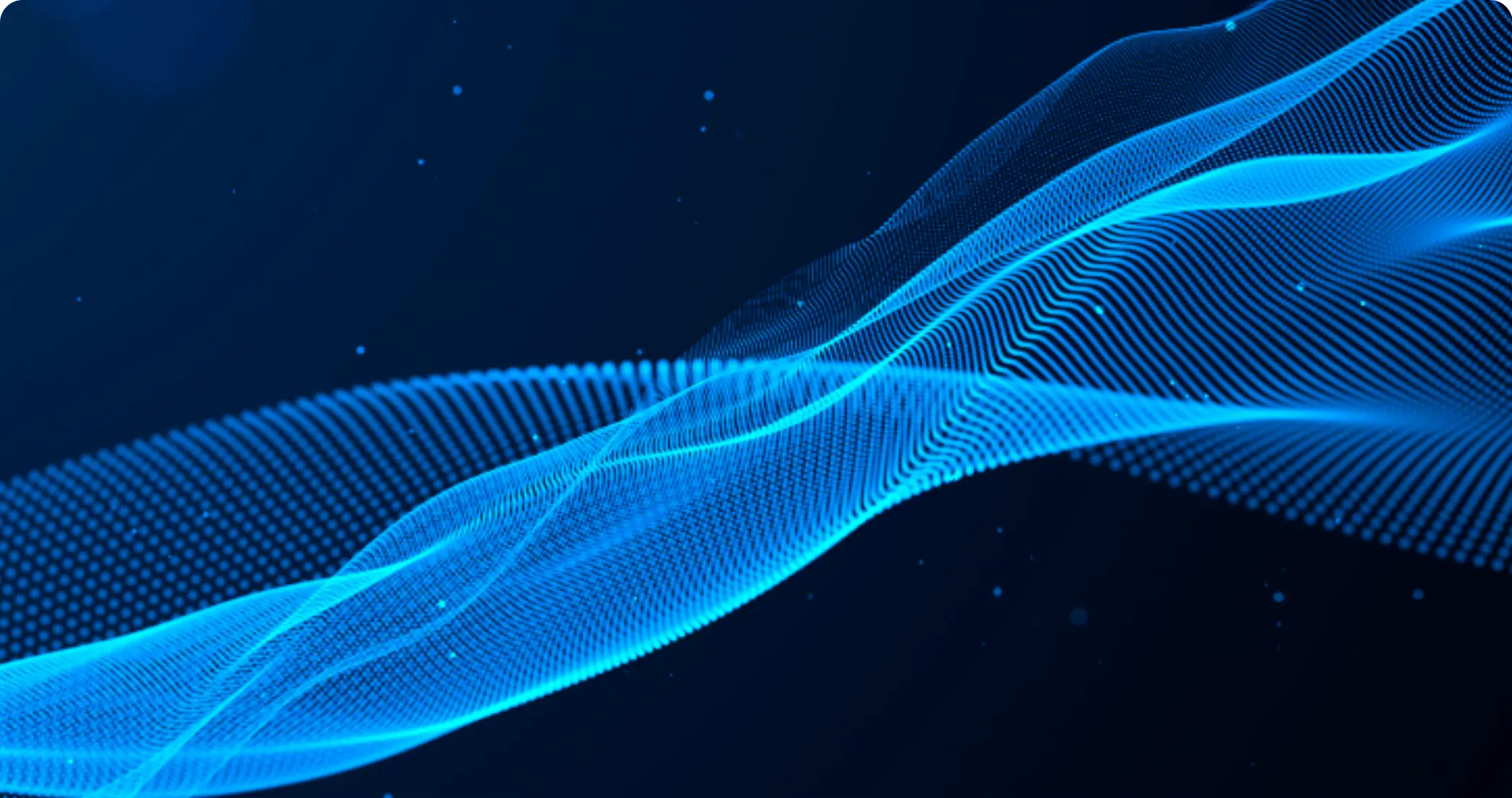
Expertise and Innovation
Backed by industry-leading technology and expertise.
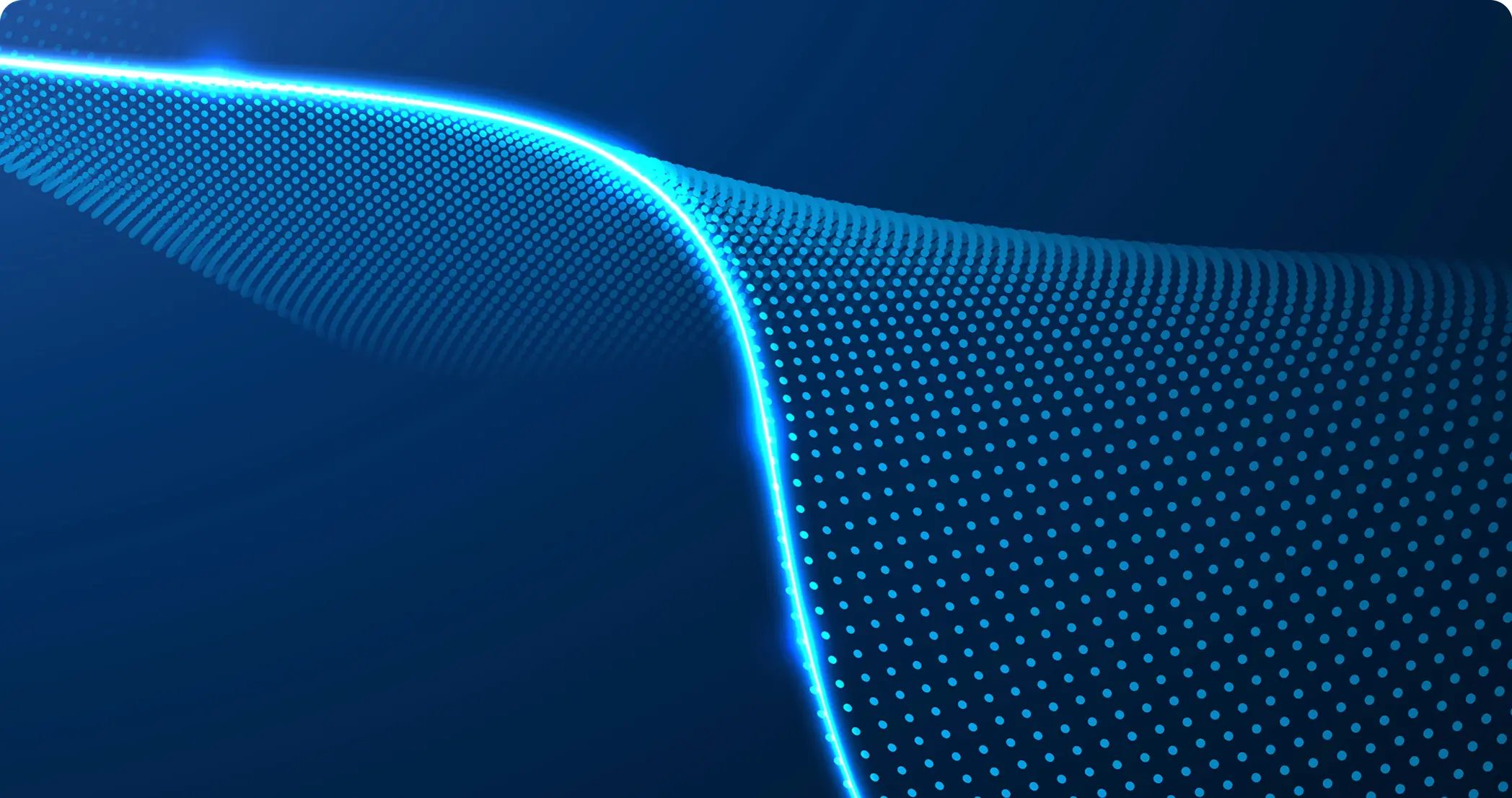
Custom Solutions
Tailored to fit your specific industrial needs.
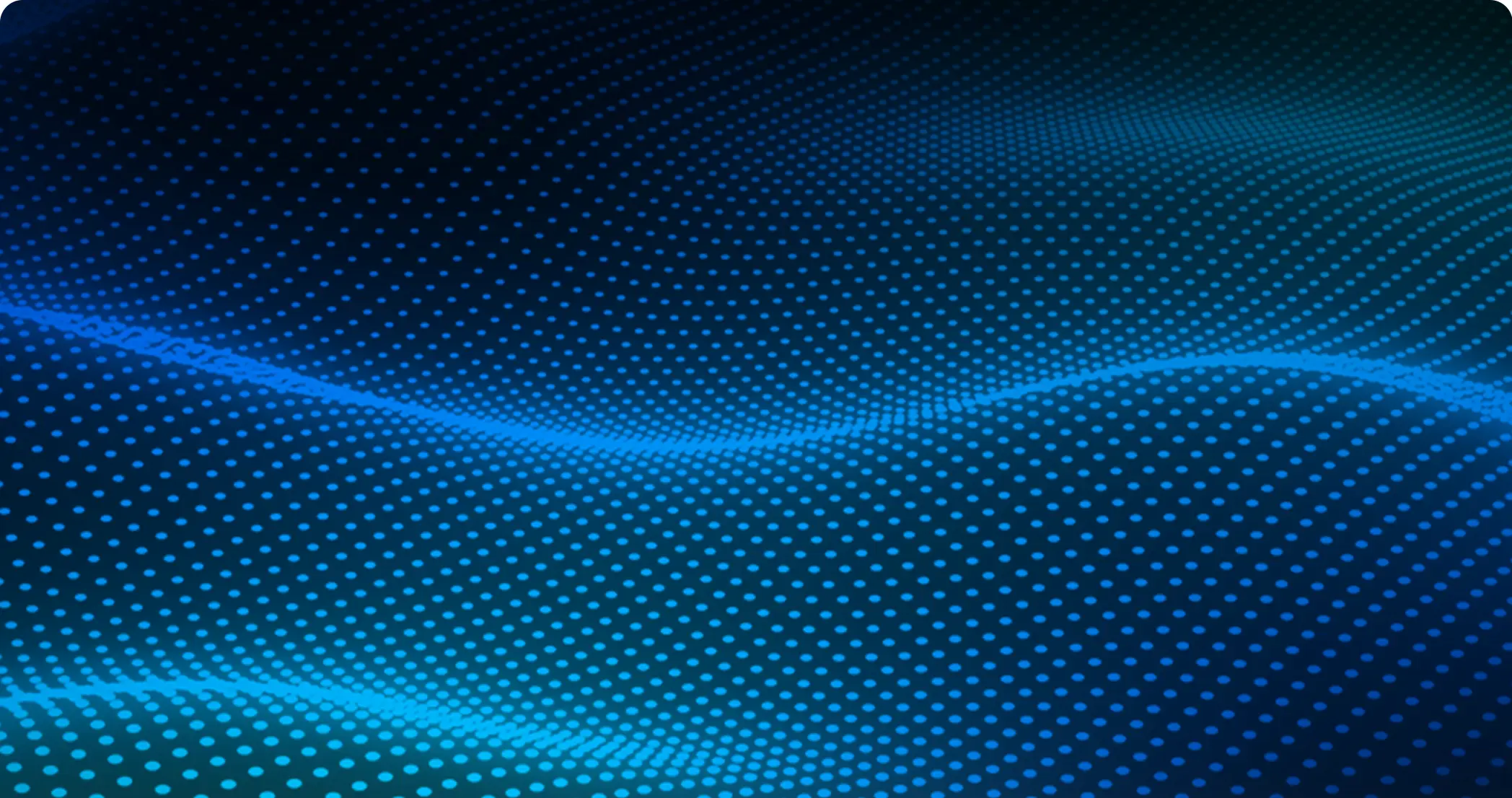
Dedicated Support
Comprehensive support every step of the way.
Benefits
Real impact of IoT and predictive maintenance that offer better business value
- Keep the right amount of spare parts by predicting exact maintenance needs, reducing overstock and last-minute orders.
- Reduce the repair times by identifying the problems early with IOT sensors to avoid costly factory shutdowns
- Increase your company ROI by reducing downtime and overall cost of maintenance so your machines last longer and work harder.
- Predict and offset any potential hazards in safety early as well as help with compliance to regulations using IoT data.
- Foster maintenance team performance by turning IOT data into automatically prioritized tasks that reduce lost production and shorten repair time.
- Schedule routine checks anytime except peak hours based on IoT insights so as not to obstruct busy production times.
Verticals we serve
Where innovation meets industry needs
By understanding industry needs and problems, Opsio’s tailored solution and expertise on IoT for predictive maintenance assist in preventing failures, reducing unplanned stoppage and optimizing asset performance.
Technology Providers
We help tech providers integrate IoT-based predictive maintenance, offering automated alerts, swift data analysis, CMMS adoption, and easy maintenance scheduling for improved client asset management.
Public Sectors
We deliver customized IoT predictive maintenance solutions for the public sector to extend infrastructure’s lifespan, improve repair timing, and increase safety across resources, transit, and public facilities.
BFSI
Opsio assists the BFSI industry to get end to end faculties of IoT solutions into the business, such as swift risk assessment, fraud detection, smart ATM operations, supply chain optimization and enhance security compliance and customer trust.
Telecom
Opsio enhances telecom with IoT predictive maintenance solutions that address poor data quality and system complexity, fostering network reliability and customer experience through data-driven proactive maintenance strategies.
Stay Ahead of the Cloud Curve
Get monthly insights on cloud transformation, DevOps strategies, and real-world case studies from the Opsio team.
Why Opsio?
Opsio: Our goal is to simplify operations with connected maintenance solutions
As a trusted collaborator with Azure, AWS, and Google Cloud, Opsio offers deep expertise and experience in building scalable IoT solutions. From preventive to predictive maintenance, we help your enterprises to move one step forward, making your operations intelligent and ensuring a reliable performance and cost-effective management cycle.
Our goal is to provide a beyond-IoT and predictive maintenance approach that is suited to your industry goals, working with your in-house team to meet technology with business priorities and long-term operational excellence.
IoT Predictive Maintenance Evolution: Your Opsio Roadmap To Success
Customer Introduction
Introductory meeting to explore needs, goals, and next steps.
Proposal
Onboarding
The shovel hits the ground through onboarding of our agreed service collaboration.
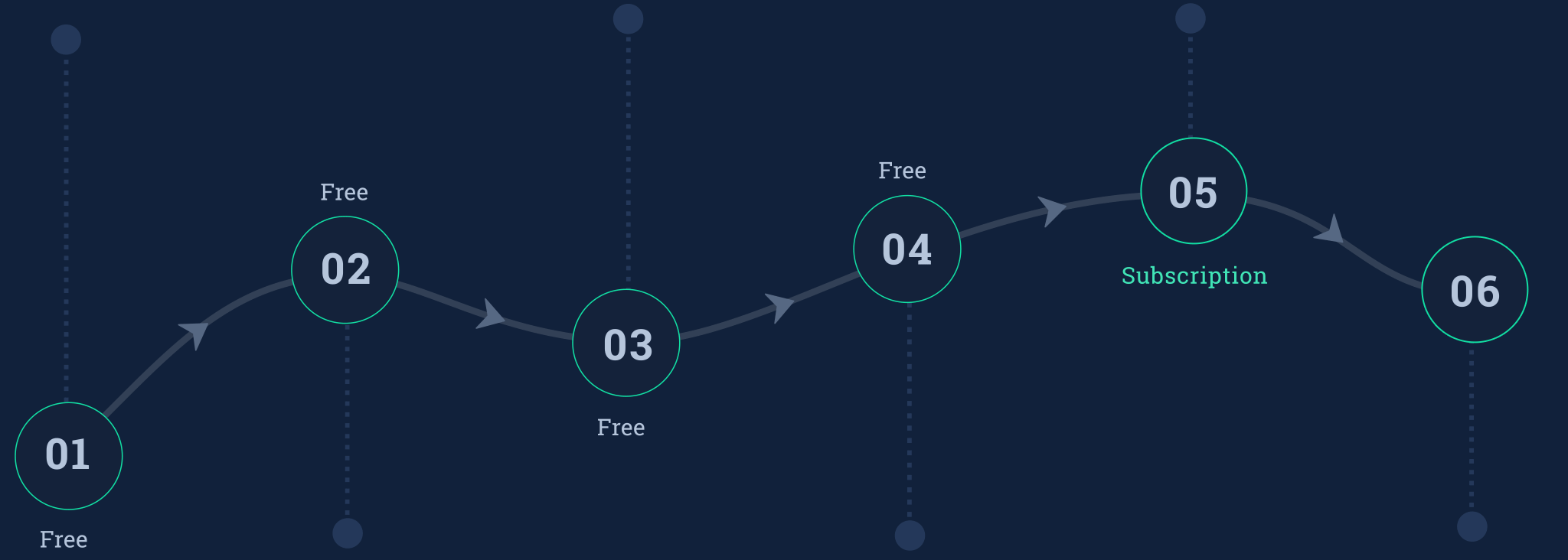
Assessment Phase
Compliance Activation
Run & Optimize
FAQ: IoT Predictive Maintenance
How to implement predictive maintenance?
“Predictive maintenance is an advanced approach to asset management that leverages data analytics, machine learning, and IoT (Internet of Things) technologies to predict when equipment failures might occur. This proactive strategy aims to optimize maintenance schedules, reduce downtime, and extend the lifespan of machinery. In this blog post, we will delve into the intricacies of implementing predictive maintenance, exploring the necessary steps, tools, and best practices to ensure a successful deployment.
Understanding Predictive Maintenance
Before diving into the implementation, it’s crucial to grasp what predictive maintenance entails. Unlike traditional maintenance strategies—such as reactive maintenance, where repairs are made after equipment fails, or preventive maintenance, which involves scheduled servicing regardless of equipment condition—predictive maintenance relies on real-time data to anticipate and mitigate potential issues before they escalate.
The core of predictive maintenance lies in its ability to analyze data from various sensors and devices attached to machinery. These sensors monitor parameters such as temperature, vibration, and pressure, transmitting this data to a central system where it is processed and analyzed. Advanced algorithms and machine learning models then interpret this data to predict potential failures, enabling maintenance teams to address issues proactively.
Laying the Groundwork for Predictive Maintenance
Implementing predictive maintenance requires a structured approach, starting with a thorough assessment of your current maintenance practices and the readiness of your organization to adopt new technologies. Begin by identifying the critical assets that would benefit the most from predictive maintenance. These are typically high-value, high-risk assets whose failure would result in significant downtime or financial loss.
Next, evaluate the existing infrastructure and data collection capabilities. Determine whether your equipment is already equipped with the necessary sensors or if additional instrumentation will be required. It’s also important to assess the quality and volume of historical maintenance data available, as this will be instrumental in training predictive models.
Investing in the Right Technology
The success of predictive maintenance hinges on the deployment of appropriate technologies. This includes IoT devices for data collection, data storage solutions, and advanced analytics platforms. IoT devices, such as vibration sensors, temperature sensors, and pressure transducers, are essential for continuous monitoring of equipment health.
Data storage solutions must be robust enough to handle the large volumes of data generated by these sensors. Cloud-based storage options are often preferred due to their scalability and ease of integration with other systems. Additionally, edge computing can be employed to process data locally, reducing latency and bandwidth usage.
For data analysis, machine learning platforms are indispensable. These platforms should offer capabilities for data preprocessing, model training, and real-time analytics. Popular tools include TensorFlow, PyTorch, and Azure Machine Learning, which provide a range of algorithms and frameworks tailored for predictive maintenance applications.
Data Integration and Management
Integrating data from various sources is a critical step in implementing predictive maintenance. This involves consolidating data from sensors, historical maintenance records, and other relevant systems into a unified platform. Data integration tools, such as Apache Kafka or Microsoft Azure IoT Hub, can facilitate this process by enabling seamless data flow between devices and analytics platforms.
Effective data management is equally important. Ensure that data is cleaned, normalized, and labeled correctly to enhance the accuracy of predictive models. Implementing a data governance framework can help maintain data quality and integrity, providing a solid foundation for analytics.
Developing Predictive Models
The heart of predictive maintenance lies in the development of accurate and reliable predictive models. This process begins with feature engineering, where relevant features are extracted from raw data. For instance, features such as mean, standard deviation, and frequency components of vibration signals can be derived to capture the characteristics of equipment behavior.
Once features are extracted, the next step is to select and train machine learning models. Commonly used algorithms include regression models, decision trees, random forests, and neural networks. The choice of algorithm depends on the complexity of the data and the specific requirements of the application.
Training predictive models requires labeled data, where historical instances of equipment failures are annotated. This allows the model to learn patterns associated with failures and make accurate predictions. Techniques such as cross-validation and hyperparameter tuning are employed to optimize model performance and prevent overfitting.
Implementing Predictive Maintenance in Operations
With predictive models in place, the next step is to integrate them into your maintenance operations. This involves setting up real-time monitoring and alert systems that notify maintenance teams of potential issues. Dashboards and visualization tools can provide insights into equipment health, enabling informed decision-making.
Establishing a feedback loop is crucial for continuous improvement. Monitor the performance of predictive models and refine them based on new data and feedback from maintenance teams. This iterative process ensures that the models remain accurate and effective over time.
Change Management and Training
Implementing predictive maintenance is not just a technological shift but also a cultural one. It requires buy-in from all stakeholders, including management, maintenance teams, and IT departments. Effective change management strategies should be employed to communicate the benefits of predictive maintenance and address any concerns.
Training is essential to equip maintenance personnel with the skills needed to interpret predictive insights and take appropriate actions. Workshops, hands-on training sessions, and online courses can help bridge the knowledge gap and foster a data-driven mindset.
Measuring Success and ROI
Finally, it’s important to measure the success of your predictive maintenance implementation. Key performance indicators (KPIs) such as reduced downtime, lower maintenance costs, and increased equipment lifespan can provide valuable insights into the effectiveness of the strategy. Conduct regular assessments and adjust your approach based on the results to maximize the return on investment (ROI).
In conclusion, implementing predictive maintenance is a multifaceted endeavor that requires careful planning, investment in technology, and a commitment to continuous improvement. By leveraging data analytics and machine learning, organizations can transform their maintenance practices, achieving greater efficiency, reliability, and cost savings.
By embracing predictive maintenance, organizations can shift from a reactive or preventive maintenance approach to a more proactive and data-driven strategy. This transition not only optimizes maintenance schedules and reduces downtime but also enhances the overall reliability and longevity of critical assets.
The foundation of predictive maintenance lies in the seamless integration of IoT devices, advanced analytics platforms, and machine learning algorithms. This sophisticated ecosystem enables organizations to harness the power of real-time data to predict and prevent equipment failures before they occur. By leveraging historical maintenance data and sensor readings, predictive models can accurately forecast potential issues, empowering maintenance teams to take preemptive action.
Successful implementation of predictive maintenance requires a holistic approach, encompassing data integration, model development, change management, and ongoing training. By investing in the right technology, developing robust predictive models, and fostering a culture of data-driven decision-making, organizations can unlock the full potential of predictive maintenance.
Ultimately, the true measure of success lies in the tangible impact of predictive maintenance on key performance indicators such as reduced downtime, lower maintenance costs, and extended equipment lifespan. By continuously monitoring performance, refining models, and adapting strategies based on results, organizations can maximize the return on investment and reap the benefits of a proactive maintenance approach.”
How does predictive maintenance work?
In the realm of modern industry, where downtime can lead to significant financial losses and operational disruptions, predictive maintenance has emerged as a game-changing strategy. But how does predictive maintenance work, and why is it becoming a cornerstone of efficient operations? This blog post delves into the intricacies of predictive maintenance, exploring its mechanisms, benefits, and the technological advancements that make it possible.
Understanding Predictive Maintenance
Predictive maintenance is a proactive approach aimed at predicting when equipment failure might occur and performing maintenance just in time to prevent it. Unlike traditional maintenance strategies, such as reactive maintenance (fixing equipment after it breaks) or preventive maintenance (routine maintenance at scheduled intervals), predictive maintenance leverages data and advanced analytics to foresee potential issues.
The Core Components of Predictive Maintenance
At the heart of predictive maintenance lies a combination of data collection, data analysis, and machine learning. These components work in unison to provide a comprehensive understanding of equipment health and performance.
1. Data Collection
The first step in predictive maintenance is data collection. Sensors are installed on machinery to monitor various parameters such as temperature, vibration, pressure, and humidity. These sensors continuously gather real-time data, which is then transmitted to a central system for analysis. The type and number of sensors used can vary depending on the complexity and criticality of the equipment being monitored.
2. Data Analysis
Once the data is collected, it undergoes rigorous analysis. Advanced analytics tools and algorithms sift through the data to identify patterns and anomalies. Historical data is also used to establish baseline performance metrics, which help in detecting deviations that may indicate potential failures. This analysis can be conducted on-site or through cloud-based platforms, offering flexibility and scalability.
3. Machine Learning and Predictive Algorithms
Machine learning plays a pivotal role in predictive maintenance. Predictive algorithms, powered by machine learning, are trained on historical and real-time data to recognize signs of wear and tear, degradation, or imminent failure. These algorithms become more accurate over time as they process more data, enabling them to provide increasingly precise predictions.
4. Condition Monitoring
Condition monitoring is an ongoing process where the health of the equipment is continuously assessed based on the data collected. This real-time monitoring allows maintenance teams to receive alerts or notifications when the equipment shows signs of potential failure. By addressing issues before they escalate, companies can avoid unexpected downtime and costly repairs.
The Benefits of Predictive Maintenance
Predictive maintenance offers a plethora of benefits that extend beyond mere cost savings. Its impact on operational efficiency, asset longevity, and overall productivity is profound.
1. Reduced Downtime
One of the most significant advantages of predictive maintenance is the reduction of unplanned downtime. By predicting when equipment is likely to fail, maintenance can be scheduled during non-peak hours or planned shutdowns, minimizing disruptions to operations.
2. Cost Efficiency
Predictive maintenance helps in optimizing maintenance schedules, reducing unnecessary maintenance activities, and preventing catastrophic failures. This approach leads to significant cost savings by avoiding expensive repairs and extending the lifespan of equipment.
3. Enhanced Safety
Predictive maintenance contributes to a safer working environment. By identifying potential issues before they lead to equipment failure, the risk of accidents and hazardous situations is minimized. This proactive approach ensures that machinery operates within safe parameters.
4. Improved Asset Management
With predictive maintenance, companies gain better control over their assets. The continuous monitoring and analysis provide valuable insights into equipment performance, enabling informed decision-making regarding repairs, replacements, and upgrades.
5. Environmental Impact
By maintaining equipment in optimal condition, predictive maintenance helps in reducing energy consumption and minimizing waste. Efficiently running machines consume less energy, leading to lower carbon emissions and a smaller environmental footprint.
Technological Advancements Driving Predictive Maintenance
The evolution of predictive maintenance is closely tied to advancements in technology. Several key technologies are instrumental in making predictive maintenance more effective and accessible.
1. Internet of Things (IoT)
The IoT revolution has significantly enhanced data collection capabilities. IoT-enabled sensors and devices can gather vast amounts of data from machinery and transmit it in real-time to centralized systems. This interconnected network of devices facilitates seamless condition monitoring and data analysis.
2. Big Data Analytics
The ability to process and analyze large datasets is crucial for predictive maintenance. Big data analytics tools enable the extraction of meaningful insights from vast amounts of information, allowing for accurate predictions and informed decision-making.
3. Artificial Intelligence (AI)
AI algorithms are the backbone of predictive maintenance. These algorithms can identify complex patterns and correlations within the data, providing actionable insights. AI-powered predictive maintenance systems continuously learn and improve, enhancing their predictive accuracy over time.
4. Cloud Computing
Cloud-based platforms offer the scalability and flexibility needed for predictive maintenance. These platforms provide the computational power required to process large datasets and run complex algorithms. Additionally, cloud computing enables remote monitoring and analysis, making predictive maintenance feasible for organizations of all sizes.
5. Edge Computing
Edge computing complements cloud computing by processing data closer to the source. This approach reduces latency and bandwidth usage, enabling real-time decision-making and faster response times. Edge computing is particularly beneficial for industries with critical equipment that requires immediate attention.
Predictive Maintenance in Action
Predictive maintenance is not just a theoretical concept; it is being successfully implemented across various industries, transforming the way maintenance is conducted.
In the manufacturing sector, predictive maintenance ensures that production lines run smoothly without unexpected interruptions. By monitoring the condition of machinery such as conveyor belts, motors, and pumps, manufacturers can schedule maintenance activities during planned downtimes, maximizing productivity.
In the energy sector, predictive maintenance is used to monitor the performance of wind turbines, solar panels, and power plants. By predicting and addressing potential issues, energy companies can maintain a steady supply of power and reduce operational costs.
In the transportation industry, predictive maintenance is applied to monitor the health of vehicles, including airplanes, trains, and trucks. This approach helps in preventing breakdowns, ensuring passenger safety, and optimizing maintenance schedules.
In the healthcare sector, predictive maintenance is used to monitor medical equipment such as MRI machines, CT scanners, and ventilators. By ensuring that these critical devices are always operational, healthcare providers can deliver uninterrupted patient care.
The Future of Predictive Maintenance
As technology continues to advance, the future of predictive maintenance looks promising. The integration of emerging technologies such as 5G, blockchain, and augmented reality (AR) is expected to further enhance predictive maintenance capabilities. These innovations will enable even more precise predictions, faster response times, and improved collaboration among maintenance teams.
Moreover, the adoption of digital twins—virtual replicas of physical assets—will revolutionize predictive maintenance. Digital twins allow for real-time simulation and analysis, providing deeper insights into equipment behavior and enabling more accurate predictions.
In conclusion, predictive maintenance represents a paradigm shift in how maintenance is approached. By leveraging data, advanced analytics, and machine learning, organizations can predict and prevent equipment failures, leading to increased efficiency, cost savings, and improved safety. As technology continues to evolve, predictive maintenance will undoubtedly play an increasingly vital role in the future of industrial operations.
Predictive Maintenance: How It Works and Why It Matters
In the realm of modern industry, where downtime can lead to significant financial losses and operational disruptions, predictive maintenance has emerged as a game-changing strategy. But how does predictive maintenance work, and why is it becoming a cornerstone of efficient operations? This blog post delves into the intricacies of predictive maintenance, exploring its mechanisms, benefits, and the technological advancements that make it possible.
Understanding Predictive Maintenance
Predictive maintenance is a proactive approach aimed at predicting when equipment failure might occur and performing maintenance just in time to prevent it. Unlike traditional maintenance strategies, such as reactive maintenance (fixing equipment after it breaks) or preventive maintenance (routine maintenance at scheduled intervals), predictive maintenance leverages data and advanced analytics to foresee potential issues.
The Core Components of Predictive Maintenance
At the heart of predictive maintenance lies a combination of data collection, data analysis, and machine learning. These components work in unison to provide a comprehensive understanding of equipment health and performance.
1. Data Collection The first step in predictive maintenance is data collection. Sensors are installed on machinery to monitor various parameters such as temperature, vibration, pressure, and humidity. These sensors continuously gather real-time data, which is then transmitted to a central system for analysis. The type and number of sensors used can vary depending on the complexity and criticality of the equipment being monitored.
2. Data Analysis Once the data is collected, it undergoes rigorous analysis. Advanced analytics tools and algorithms sift through the data to identify patterns and anomalies. Historical data is also used to establish baseline performance metrics, which help in detecting deviations that may indicate potential failures. This analysis can be conducted on-site or through cloud-based platforms, offering flexibility and scalability.
3. Machine Learning and Predictive Algorithms Machine learning plays a pivotal role in predictive maintenance. Predictive algorithms, powered by machine learning, are trained on historical and real-time data to recognize signs of wear and tear, degradation, or imminent failure. These algorithms become more accurate over time as they process more data, enabling them to provide increasingly precise predictions.
4. Condition Monitoring Condition monitoring is an ongoing process where the health of the equipment is continuously assessed based on the data collected. This real-time monitoring allows maintenance teams to receive alerts or notifications when the equipment shows signs of potential failure. By addressing issues before they escalate, companies can avoid unexpected downtime and costly repairs.
The Benefits of Predictive Maintenance
Predictive maintenance offers a plethora of benefits that extend beyond mere cost savings. Its impact on operational efficiency, asset longevity, and overall productivity is profound.
1. Reduced Downtime One of the most significant advantages of predictive maintenance is the reduction of unplanned downtime. By predicting when equipment is likely to fail, maintenance can be scheduled during non-peak hours or planned shutdowns, minimizing disruptions to operations.
2. Cost Efficiency Predictive maintenance helps in optimizing maintenance schedules, reducing unnecessary maintenance activities, and preventing catastrophic failures. This approach leads to significant cost savings by avoiding expensive repairs and extending the lifespan of equipment.
3. Enhanced Safety Predictive maintenance contributes to a safer working environment. By identifying potential issues before they lead to equipment failure, the risk of accidents and hazardous situations is minimized. This proactive approach ensures that machinery operates within safe parameters.
4. Improved Asset Management With predictive maintenance, companies gain better control over their assets. The continuous monitoring and analysis provide valuable insights into equipment performance, enabling informed decision-making regarding repairs, replacements, and upgrades.
5. Environmental Impact By maintaining equipment in optimal condition, predictive maintenance helps in reducing energy consumption and minimizing waste. Efficiently running machines consume less energy, leading to lower carbon emissions and a smaller environmental footprint.
Technological Advancements Driving Predictive Maintenance
The evolution of predictive maintenance is closely tied to advancements in technology. Several key technologies are instrumental in making predictive maintenance more effective and accessible.
1. Internet of Things (IoT) The IoT revolution has significantly enhanced data collection capabilities. IoT-enabled sensors and devices can gather vast amounts of data from machinery and transmit it in real-time to centralized systems. This interconnected network of devices facilitates seamless condition monitoring and data analysis.
2. Big Data Analytics The ability to process and analyze large datasets is crucial for predictive maintenance. Big data analytics tools enable the extraction of meaningful insights from vast amounts of information, allowing for accurate predictions and informed decision-making.
3. Artificial Intelligence (AI) AI algorithms are the backbone of predictive maintenance. These algorithms can identify complex patterns and correlations within the data, providing actionable insights. AI-powered predictive maintenance systems continuously learn and improve, enhancing their predictive accuracy over time.
4. Cloud Computing Cloud-based platforms offer the scalability and flexibility needed for predictive maintenance. These platforms provide the computational power required to process large datasets and run complex algorithms. Additionally, cloud computing enables remote monitoring and analysis, making predictive maintenance feasible for organizations of all sizes.
5. Edge Computing Edge computing complements cloud computing by processing data closer to the source. This approach reduces latency and bandwidth usage, enabling real-time decision-making and faster response times. Edge computing is particularly beneficial for industries with critical equipment that requires immediate attention.
Predictive Maintenance in Action
Predictive maintenance is not just a theoretical concept; it is being successfully implemented across various industries, transforming the way maintenance is conducted.
In the manufacturing sector, predictive maintenance ensures that production lines run smoothly without unexpected interruptions. By monitoring the condition of machinery such as conveyor belts, motors, and pumps, manufacturers can schedule maintenance activities during planned downtimes, maximizing productivity.
In the energy sector, predictive maintenance is used to monitor the performance of wind turbines, solar panels, and power plants. By predicting and addressing potential issues, energy companies can maintain a steady supply of power and reduce operational costs.
In the transportation industry, predictive maintenance is applied to monitor the health of vehicles, including airplanes, trains, and trucks. This approach helps in preventing breakdowns, ensuring passenger safety, and optimizing maintenance schedules.
In the healthcare sector, predictive maintenance is used to monitor medical equipment such as MRI machines, CT scanners, and ventilators. By ensuring that these critical devices are always operational, healthcare providers can deliver uninterrupted patient care.
The Future of Predictive Maintenance
As technology continues to advance, the future of predictive maintenance looks promising. The integration of emerging technologies such as 5G, blockchain, and augmented reality (AR) is expected to further enhance predictive maintenance capabilities. These innovations will enable even more precise predictions, faster response times, and improved collaboration among maintenance teams.
Moreover, the adoption of digital twins—virtual replicas of physical assets—will revolutionize predictive maintenance. Digital twins allow for real-time simulation and analysis, providing deeper insights into equipment behavior and enabling more accurate predictions.
In conclusion, predictive maintenance represents a paradigm shift in how maintenance is approached. By leveraging data, advanced analytics, and machine learning, organizations can predict and prevent equipment failures, leading to increased efficiency, cost savings, and improved safety. As technology continues to evolve, predictive maintenance will undoubtedly play an increasingly vital role in the future of industrial operations.”
What is the purpose of predictive maintenance?
In today’s fast-paced industrial landscape, the efficiency and reliability of machinery and equipment are paramount. Unplanned downtimes can lead to significant financial losses and operational disruptions. This is where predictive maintenance comes into play. The purpose of predictive maintenance is to foresee potential equipment failures and address them before they lead to costly downtimes. By leveraging advanced technologies and data analytics, predictive maintenance aims to enhance the longevity and performance of machinery, ultimately benefiting businesses in multiple ways.
The Core Concept of Predictive Maintenance
Predictive maintenance revolves around the idea of predicting when equipment failure might occur and preemptively addressing the issue. Unlike reactive maintenance, which deals with equipment after it breaks down, or preventive maintenance, which involves regular, scheduled maintenance, predictive maintenance is based on the actual condition of the equipment. It uses real-time data, historical data, and sophisticated algorithms to predict future failures.
The Role of Data in Predictive Maintenance
Data is the backbone of predictive maintenance. Sensors and IoT devices are installed on machinery to continuously monitor various parameters such as temperature, vibration, noise, and pressure. This data is then transmitted to a central system where it is analyzed using machine learning algorithms and AI models. By identifying patterns and anomalies in the data, these systems can predict potential failures with a high degree of accuracy.
For instance, a sudden spike in vibration levels might indicate that a machine bearing is about to fail. By detecting this anomaly early, maintenance teams can intervene before the bearing actually fails, preventing unplanned downtime and extending the machine’s lifespan.
Benefits of Predictive Maintenance
Predictive maintenance offers numerous benefits that go beyond just preventing equipment failure. One of the most significant advantages is cost savings. By addressing issues before they escalate into major problems, companies can avoid expensive repairs and replacements. Additionally, predictive maintenance reduces the need for unnecessary maintenance activities, optimizing the use of resources and labor.
Another key benefit is improved safety. Equipment failures can pose serious safety risks to workers. By ensuring that machinery is always in optimal condition, predictive maintenance helps create a safer working environment. Moreover, it enhances operational efficiency by minimizing downtime and ensuring that production processes run smoothly.
Implementing Predictive Maintenance
Implementing predictive maintenance requires a strategic approach. It begins with identifying the critical assets that need monitoring. These are typically the machines and equipment that are vital to the production process and whose failure would have significant consequences.
Next, the necessary sensors and IoT devices must be installed to collect real-time data. This data needs to be integrated into a centralized system where it can be analyzed. The choice of software and analytics tools is crucial, as they must be capable of processing large volumes of data and providing actionable insights.
Training and collaboration are also essential. Maintenance teams need to be trained in using the new technologies and interpreting the data. Collaboration between IT, operations, and maintenance departments is vital to ensure a smooth implementation and ongoing success.
Challenges of Predictive Maintenance
While the benefits of predictive maintenance are clear, implementing it is not without challenges. One of the primary challenges is the initial cost. Installing sensors, setting up data analytics systems, and training staff require a significant investment. However, this cost is often offset by the long-term savings achieved through reduced downtime and maintenance expenses.
Data management is another challenge. The sheer volume of data generated by sensors can be overwhelming. Ensuring that this data is accurate, relevant, and properly analyzed requires robust data management practices and advanced analytics tools.
Additionally, there can be resistance to change within organizations. Shifting from traditional maintenance practices to a predictive maintenance model requires a cultural change. It is essential to communicate the benefits clearly and involve all stakeholders in the process to overcome this resistance.
Future of Predictive Maintenance
The future of predictive maintenance looks promising as technology continues to evolve. Advances in AI and machine learning are making predictive models more accurate and reliable. The integration of predictive maintenance with other Industry 4.0 technologies, such as digital twins and augmented reality, is opening up new possibilities for enhancing equipment maintenance and management.
Moreover, as more industries recognize the value of predictive maintenance, it is likely to become a standard practice across various sectors. From manufacturing and energy to transportation and healthcare, the potential applications of predictive maintenance are vast and varied.
In conclusion, the purpose of predictive maintenance is to leverage data and technology to foresee and prevent equipment failures. By doing so, it enhances operational efficiency, reduces costs, and improves safety. While there are challenges to implementation, the long-term benefits make predictive maintenance a worthwhile investment for any forward-thinking organization. As technology continues to advance, the capabilities and applications of predictive maintenance will only expand, making it an integral part of modern industrial practices.
Understanding the Purpose of Predictive Maintenance
In today’s fast-paced industrial landscape, the efficiency and reliability of machinery and equipment are paramount. Unplanned downtimes can lead to significant financial losses and operational disruptions. This is where predictive maintenance comes into play. The purpose of predictive maintenance is to foresee potential equipment failures and address them before they lead to costly downtimes. By leveraging advanced technologies and data analytics, predictive maintenance aims to enhance the longevity and performance of machinery, ultimately benefiting businesses in multiple ways.
The Core Concept of Predictive Maintenance
Predictive maintenance revolves around the idea of predicting when equipment failure might occur and preemptively addressing the issue. Unlike reactive maintenance, which deals with equipment after it breaks down, or preventive maintenance, which involves regular, scheduled maintenance, predictive maintenance is based on the actual condition of the equipment. It uses real-time data, historical data, and sophisticated algorithms to predict future failures.
The Role of Data in Predictive Maintenance
Data is the backbone of predictive maintenance. Sensors and IoT devices are installed on machinery to continuously monitor various parameters such as temperature, vibration, noise, and pressure. This data is then transmitted to a central system where it is analyzed using machine learning algorithms and AI models. By identifying patterns and anomalies in the data, these systems can predict potential failures with a high degree of accuracy.
For instance, a sudden spike in vibration levels might indicate that a machine bearing is about to fail. By detecting this anomaly early, maintenance teams can intervene before the bearing actually fails, preventing unplanned downtime and extending the machine’s lifespan.
Benefits of Predictive Maintenance
Predictive maintenance offers numerous benefits that go beyond just preventing equipment failure. One of the most significant advantages is cost savings. By addressing issues before they escalate into major problems, companies can avoid expensive repairs and replacements. Additionally, predictive maintenance reduces the need for unnecessary maintenance activities, optimizing the use of resources and labor.
Another key benefit is improved safety. Equipment failures can pose serious safety risks to workers. By ensuring that machinery is always in optimal condition, predictive maintenance helps create a safer working environment. Moreover, it enhances operational efficiency by minimizing downtime and ensuring that production processes run smoothly.
Implementing Predictive Maintenance
Implementing predictive maintenance requires a strategic approach. It begins with identifying the critical assets that need monitoring. These are typically the machines and equipment that are vital to the production process and whose failure would have significant consequences.
Next, the necessary sensors and IoT devices must be installed to collect real-time data. This data needs to be integrated into a centralized system where it can be analyzed. The choice of software and analytics tools is crucial, as they must be capable of processing large volumes of data and providing actionable insights.
Training and collaboration are also essential. Maintenance teams need to be trained in using the new technologies and interpreting the data. Collaboration between IT, operations, and maintenance departments is vital to ensure a smooth implementation and ongoing success.
Challenges of Predictive Maintenance
While the benefits of predictive maintenance are clear, implementing it is not without challenges. One of the primary challenges is the initial cost. Installing sensors, setting up data analytics systems, and training staff require a significant investment. However, this cost is often offset by the long-term savings achieved through reduced downtime and maintenance expenses.
Data management is another challenge. The sheer volume of data generated by sensors can be overwhelming. Ensuring that this data is accurate, relevant, and properly analyzed requires robust data management practices and advanced analytics tools.
Additionally, there can be resistance to change within organizations. Shifting from traditional maintenance practices to a predictive maintenance model requires a cultural change. It is essential to communicate the benefits clearly and involve all stakeholders in the process to overcome this resistance.
Future of Predictive Maintenance
The future of predictive maintenance looks promising as technology continues to evolve. Advances in AI and machine learning are making predictive models more accurate and reliable. The integration of predictive maintenance with other Industry 4.0 technologies, such as digital twins and augmented reality, is opening up new possibilities for enhancing equipment maintenance and management.
Moreover, as more industries recognize the value of predictive maintenance, it is likely to become a standard practice across various sectors. From manufacturing and energy to transportation and healthcare, the potential applications of predictive maintenance are vast and varied.
Case Studies and Real-World Applications
To further understand the transformative potential of predictive maintenance, let’s look at some real-world applications and case studies.
Manufacturing Industry
In the manufacturing sector, companies like General Electric (GE) have successfully implemented predictive maintenance to monitor their industrial machinery. By using sensors to collect data on machine performance, GE can predict when a component is likely to fail and schedule maintenance accordingly. This has resulted in a significant reduction in unplanned downtimes and maintenance costs.
Energy Sector
In the energy sector, predictive maintenance is being used to monitor wind turbines. Companies like Siemens Gamesa Renewable Energy have equipped their turbines with sensors that track parameters such as vibration and temperature. By analyzing this data, they can predict potential failures and perform maintenance before a breakdown occurs, ensuring continuous energy production and reducing repair costs.
Transportation Industry
The transportation industry also benefits from predictive maintenance. For instance, airlines use predictive maintenance to monitor the health of aircraft engines. By analyzing data from engine sensors, airlines can predict when an engine component is likely to fail and perform maintenance before it leads to a flight delay or cancellation. This not only improves operational efficiency but also enhances passenger safety.
Healthcare Sector
In the healthcare sector, predictive maintenance is used to ensure the reliability of critical medical equipment. Hospitals use sensors to monitor the performance of devices such as MRI machines and ventilators. By predicting potential failures, hospitals can perform maintenance proactively, ensuring that these vital machines are always available for patient care.
Conclusion
In conclusion, the purpose of predictive maintenance is to leverage data and technology to foresee and prevent equipment failures. By doing so, it enhances operational efficiency, reduces costs, and improves safety. While there are challenges to implementation, the long-term benefits make predictive maintenance a worthwhile investment for any forward-thinking organization. As technology continues to advance, the capabilities and applications of predictive maintenance will only expand, making it an integral part of modern industrial practices.
The real-world applications and case studies demonstrate the tangible benefits of predictive maintenance across various industries. By adopting predictive maintenance, companies can not only improve their bottom line but also enhance safety, efficiency, and reliability. As we move towards a more connected and data-driven future, predictive maintenance will undoubtedly play a crucial role in shaping the industrial landscape.”
What is predictive maintenance?
In today’s fast-paced industrial landscape, the quest for operational efficiency and cost reduction is more critical than ever. One of the most transformative approaches to achieving these goals is predictive maintenance. But what exactly is predictive maintenance, and why is it garnering so much attention across various sectors?
Predictive maintenance, often abbreviated as PdM, is a proactive maintenance strategy that utilizes data analysis tools and techniques to detect anomalies and predict equipment failures before they occur. Unlike traditional maintenance methods, which can be reactive (fixing problems after they arise) or preventive (scheduled maintenance regardless of equipment condition), predictive maintenance aims to optimize the timing of maintenance tasks to minimize downtime and extend the lifespan of assets.
At its core, predictive maintenance leverages advanced technologies such as the Internet of Things (IoT), machine learning, and big data analytics. These technologies work in tandem to collect real-time data from equipment sensors, analyze this data to identify patterns, and predict potential failures. This data-driven approach not only enhances the reliability of machinery but also significantly reduces maintenance costs and improves overall operational efficiency.
One of the key components of predictive maintenance is the use of IoT sensors. These sensors are embedded in machinery and continuously monitor various parameters such as temperature, vibration, pressure, and more. The collected data is then transmitted to a central system where it is analyzed using sophisticated algorithms. Machine learning models, a subset of artificial intelligence, play a crucial role in this analysis. These models are trained on historical data to recognize normal operating conditions and identify deviations that may indicate an impending failure.
The benefits of predictive maintenance are manifold. First and foremost, it helps in reducing unplanned downtime. Unplanned downtime can be costly and disruptive, leading to lost productivity and revenue. By predicting when a piece of equipment is likely to fail, maintenance can be scheduled during planned downtime, ensuring minimal disruption to operations.
Moreover, predictive maintenance extends the lifespan of assets. Regular maintenance, guided by predictive insights, ensures that machinery operates within optimal parameters, reducing wear and tear. This not only extends the useful life of the equipment but also improves its efficiency and performance.
Another significant advantage is cost savings. Traditional maintenance approaches often lead to over-maintenance or under-maintenance. Over-maintenance results in unnecessary costs, while under-maintenance can lead to catastrophic failures and expensive repairs. Predictive maintenance strikes the perfect balance by providing maintenance teams with accurate insights into when and where maintenance is needed, optimizing resource allocation and reducing costs.
In addition to these tangible benefits, predictive maintenance also enhances safety. Equipment failures can pose significant safety risks, especially in industries such as manufacturing, oil and gas, and transportation. By predicting and preventing failures, predictive maintenance helps in mitigating these risks, ensuring a safer working environment for employees.
The implementation of predictive maintenance, however, is not without its challenges. One of the primary challenges is the initial investment in IoT sensors, data analytics tools, and skilled personnel. While the long-term benefits far outweigh these initial costs, organizations need to be prepared for the upfront investment. Additionally, the integration of predictive maintenance into existing systems and workflows can be complex and requires careful planning and execution.
Another challenge is data management. The sheer volume of data generated by IoT sensors can be overwhelming. Organizations need robust data management systems to collect, store, and analyze this data effectively. Ensuring data quality and accuracy is also crucial, as inaccurate data can lead to incorrect predictions and suboptimal maintenance decisions.
Despite these challenges, the adoption of predictive maintenance is on the rise, driven by the compelling benefits it offers. Industries such as manufacturing, energy, transportation, and healthcare are increasingly embracing predictive maintenance to enhance operational efficiency, reduce costs, and improve safety.
In the manufacturing sector, for instance, predictive maintenance is being used to monitor critical machinery such as conveyor belts, motors, and pumps. By analyzing data from these machines, manufacturers can predict failures and schedule maintenance during planned downtimes, minimizing production disruptions.
In the energy sector, predictive maintenance is being applied to monitor equipment such as turbines, transformers, and pipelines. By predicting failures, energy companies can prevent costly outages and ensure a reliable supply of energy.
In the transportation sector, predictive maintenance is being used to monitor vehicles and infrastructure. For example, railway companies are using predictive maintenance to monitor tracks and trains, ensuring safe and reliable operations.
In the healthcare sector, predictive maintenance is being applied to medical equipment such as MRI machines, CT scanners, and ventilators. By predicting failures, healthcare providers can ensure that critical equipment is always available for patient care.
The future of predictive maintenance looks promising, with advancements in IoT, machine learning, and data analytics paving the way for even more sophisticated and accurate predictions. As technology continues to evolve, predictive maintenance will become an integral part of asset management and operational efficiency strategies across various industries.
In conclusion, predictive maintenance represents a significant shift from traditional maintenance approaches. By leveraging advanced technologies to predict and prevent equipment failures, it offers numerous benefits, including reduced downtime, extended asset lifespan, cost savings, and enhanced safety. While the implementation of predictive maintenance comes with its challenges, the long-term benefits make it a worthwhile investment for organizations aiming to optimize their operations and stay competitive in today’s dynamic industrial landscape.
Predictive Maintenance: Revolutionizing Asset Management and Operational Efficiency
In today’s fast-paced industrial landscape, the quest for operational efficiency and cost reduction is more critical than ever. One of the most transformative approaches to achieving these goals is predictive maintenance. But what exactly is predictive maintenance, and why is it garnering so much attention across various sectors?
Predictive maintenance, often abbreviated as PdM, is a proactive maintenance strategy that utilizes data analysis tools and techniques to detect anomalies and predict equipment failures before they occur. Unlike traditional maintenance methods, which can be reactive (fixing problems after they arise) or preventive (scheduled maintenance regardless of equipment condition), predictive maintenance aims to optimize the timing of maintenance tasks to minimize downtime and extend the lifespan of assets.
At its core, predictive maintenance leverages advanced technologies such as the Internet of Things (IoT), machine learning, and big data analytics. These technologies work in tandem to collect real-time data from equipment sensors, analyze this data to identify patterns, and predict potential failures. This data-driven approach not only enhances the reliability of machinery but also significantly reduces maintenance costs and improves overall operational efficiency.
One of the key components of predictive maintenance is the use of IoT sensors. These sensors are embedded in machinery and continuously monitor various parameters such as temperature, vibration, pressure, and more. The collected data is then transmitted to a central system where it is analyzed using sophisticated algorithms. Machine learning models, a subset of artificial intelligence, play a crucial role in this analysis. These models are trained on historical data to recognize normal operating conditions and identify deviations that may indicate an impending failure.
The benefits of predictive maintenance are manifold. First and foremost, it helps in reducing unplanned downtime. Unplanned downtime can be costly and disruptive, leading to lost productivity and revenue. By predicting when a piece of equipment is likely to fail, maintenance can be scheduled during planned downtime, ensuring minimal disruption to operations.
Moreover, predictive maintenance extends the lifespan of assets. Regular maintenance, guided by predictive insights, ensures that machinery operates within optimal parameters, reducing wear and tear. This not only extends the useful life of the equipment but also improves its efficiency and performance.
Another significant advantage is cost savings. Traditional maintenance approaches often lead to over-maintenance or under-maintenance. Over-maintenance results in unnecessary costs, while under-maintenance can lead to catastrophic failures and expensive repairs. Predictive maintenance strikes the perfect balance by providing maintenance teams with accurate insights into when and where maintenance is needed, optimizing resource allocation and reducing costs.
In addition to these tangible benefits, predictive maintenance also enhances safety. Equipment failures can pose significant safety risks, especially in industries such as manufacturing, oil and gas, and transportation. By predicting and preventing failures, predictive maintenance helps in mitigating these risks, ensuring a safer working environment for employees.
The implementation of predictive maintenance, however, is not without its challenges. One of the primary challenges is the initial investment in IoT sensors, data analytics tools, and skilled personnel. While the long-term benefits far outweigh these initial costs, organizations need to be prepared for the upfront investment. Additionally, the integration of predictive maintenance into existing systems and workflows can be complex and requires careful planning and execution.
Another challenge is data management. The sheer volume of data generated by IoT sensors can be overwhelming. Organizations need robust data management systems to collect, store, and analyze this data effectively. Ensuring data quality and accuracy is also crucial, as inaccurate data can lead to incorrect predictions and suboptimal maintenance decisions.
Despite these challenges, the adoption of predictive maintenance is on the rise, driven by the compelling benefits it offers. Industries such as manufacturing, energy, transportation, and healthcare are increasingly embracing predictive maintenance to enhance operational efficiency, reduce costs, and improve safety.
In the manufacturing sector, for instance, predictive maintenance is being used to monitor critical machinery such as conveyor belts, motors, and pumps. By analyzing data from these machines, manufacturers can predict failures and schedule maintenance during planned downtimes, minimizing production disruptions.
In the energy sector, predictive maintenance is being applied to monitor equipment such as turbines, transformers, and pipelines. By predicting failures, energy companies can prevent costly outages and ensure a reliable supply of energy.
In the transportation sector, predictive maintenance is being used to monitor vehicles and infrastructure. For example, railway companies are using predictive maintenance to monitor tracks and trains, ensuring safe and reliable operations.
In the healthcare sector, predictive maintenance is being applied to medical equipment such as MRI machines, CT scanners, and ventilators. By predicting failures, healthcare providers can ensure that critical equipment is always available for patient care.
The future of predictive maintenance looks promising, with advancements in IoT, machine learning, and data analytics paving the way for even more sophisticated and accurate predictions. As technology continues to evolve, predictive maintenance will become an integral part of asset management and operational efficiency strategies across various industries.
The integration of predictive maintenance with other emerging technologies like digital twins and augmented reality (AR) further amplifies its potential. Digital twins create a virtual replica of physical assets, allowing for real-time simulation and analysis. This can enhance predictive maintenance by providing a comprehensive view of asset health. AR, on the other hand, can assist maintenance personnel by overlaying digital information onto physical equipment, guiding them through complex maintenance tasks and ensuring accuracy.
Moreover, the role of predictive maintenance in sustainability cannot be overlooked. By optimizing maintenance schedules and reducing unnecessary replacements, it contributes to resource conservation and reduces waste. This aligns with the growing emphasis on sustainable practices in industries worldwide.
In conclusion, predictive maintenance represents a significant shift from traditional maintenance approaches. By leveraging advanced technologies to predict and prevent equipment failures, it offers numerous benefits, including reduced downtime, extended asset lifespan, cost savings, and enhanced safety. While the implementation of predictive maintenance comes with its challenges, the long-term benefits make it a worthwhile investment for organizations aiming to optimize their operations and stay competitive in today’s dynamic industrial landscape. As technology continues to advance, the scope and impact of predictive maintenance will only grow, solidifying its role as a cornerstone of modern asset management and operational efficiency strategies.”
How to implement machine learning for predictive maintenance?
Predictive maintenance has emerged as a game-changer in various industries, offering the potential to foresee equipment failures and optimize maintenance schedules. Leveraging machine learning (ML) for predictive maintenance can significantly reduce downtime, lower maintenance costs, and extend the lifespan of machinery. This comprehensive guide will walk you through the intricacies of implementing machine learning for predictive maintenance, ensuring you harness its full potential.
Understanding Predictive Maintenance
Predictive maintenance refers to the practice of predicting when equipment will fail so that maintenance can be performed just in time to avoid downtime. Unlike preventive maintenance, which relies on scheduled maintenance activities, predictive maintenance uses data-driven insights to determine the exact moment when a machine is likely to fail. This approach minimizes unnecessary maintenance and maximizes equipment uptime.
The Role of Machine Learning in Predictive Maintenance
Machine learning algorithms excel at identifying patterns and anomalies in vast datasets. In the context of predictive maintenance, ML models analyze historical and real-time data from sensors and other sources to predict equipment failures. By continuously learning from new data, these models become more accurate over time, providing increasingly reliable predictions.
Steps to Implement Machine Learning for Predictive Maintenance
1. Data Collection and Preprocessing
The foundation of any machine learning project is data. For predictive maintenance, relevant data can come from various sources, including IoT sensors, maintenance logs, and operational records. It’s crucial to collect high-quality data that accurately reflects the condition and performance of your equipment.
Once collected, data preprocessing is essential to clean and normalize the data. This step involves handling missing values, removing outliers, and transforming data into a format suitable for machine learning algorithms. Feature engineering, where you create new features from existing data, can also enhance the predictive power of your models.
2. Choosing the Right Machine Learning Algorithm
Several machine learning algorithms can be employed for predictive maintenance, each with its strengths and weaknesses. Some popular choices include:
Regression Algorithms: Useful for predicting continuous values, such as the remaining useful life (RUL) of equipment.
Classification Algorithms: Ideal for categorizing equipment into different states, such as normal or faulty.
Anomaly Detection Algorithms: Effective for identifying unusual patterns that may indicate impending failures.
The choice of algorithm depends on the specific requirements of your predictive maintenance application. Experimenting with multiple algorithms and evaluating their performance is often necessary to find the best fit.
3. Model Training and Evaluation
Once you’ve selected an algorithm, the next step is to train your model using historical data. Splitting the data into training and validation sets allows you to assess the model’s performance on unseen data. Key evaluation metrics for predictive maintenance models include accuracy, precision, recall, and the F1 score.
It’s essential to regularly retrain your model with new data to ensure it remains accurate and relevant. Continuous monitoring and evaluation help identify any drifts in model performance, allowing for timely adjustments.
4. Integration with Maintenance Systems
For predictive maintenance to be effective, machine learning models must be integrated with your existing maintenance systems. This integration enables seamless communication between the predictive insights generated by the models and the maintenance actions taken by your team.
Implementing a robust data pipeline ensures that data flows smoothly from sensors to the machine learning models and finally to the maintenance systems. Real-time data processing capabilities are crucial for timely predictions and interventions.
5. Visualization and Interpretation
Visualizing the predictions and insights generated by your machine learning models can greatly enhance their usability. Dashboards and visualization tools provide a clear and intuitive way for maintenance teams to interpret the data and make informed decisions.
Interactive visualizations that allow users to drill down into specific equipment or time periods can uncover valuable insights. Combining predictive maintenance data with other operational metrics can also provide a holistic view of your equipment’s performance.
6. Feedback Loop and Continuous Improvement
Implementing a feedback loop is vital for the continuous improvement of your predictive maintenance system. Maintenance actions taken based on model predictions should be recorded and fed back into the system. This feedback helps refine the models and improve their accuracy over time.
Encouraging collaboration between data scientists, maintenance engineers, and operations teams fosters a culture of continuous learning and improvement. Regularly reviewing the performance of your predictive maintenance system and incorporating user feedback ensures it remains effective and aligned with your business goals.
Challenges and Considerations
Implementing machine learning for predictive maintenance is not without its challenges. Data quality and availability can significantly impact the performance of your models. Ensuring that your data is representative of all possible operating conditions is crucial for accurate predictions.
Scalability is another consideration. As the volume of data and the number of assets increase, your predictive maintenance system must be able to handle the additional load. Cloud-based solutions and edge computing can help address scalability challenges.
Finally, it’s important to consider the human element. Change management and training are essential to ensure that maintenance teams understand and trust the predictions generated by the machine learning models. Clear communication and demonstrating the value of predictive maintenance can help drive adoption and success.
By following these steps and addressing the associated challenges, you can effectively implement machine learning for predictive maintenance, unlocking significant benefits for your organization. From reducing downtime to optimizing maintenance schedules, predictive maintenance powered by machine learning is poised to revolutionize the way we manage and maintain equipment.
Additional Insights:
One key aspect to consider when implementing machine learning for predictive maintenance is the importance of interpretability. While machine learning models can provide accurate predictions, understanding how these predictions are generated is crucial for gaining trust and buy-in from maintenance teams. Transparent models that offer explanations for their decisions can help users understand the reasoning behind maintenance recommendations.
Moreover, incorporating domain knowledge into the machine learning process can further enhance the accuracy and relevance of predictive maintenance models. Subject matter experts can provide valuable insights into the intricacies of equipment behavior and failure modes, guiding the feature selection process and model development.
Collaboration between data scientists, maintenance engineers, and domain experts is essential for bridging the gap between data-driven insights and practical maintenance actions. By fostering a multidisciplinary approach to predictive maintenance, organizations can leverage the strengths of each team member to create a comprehensive and effective predictive maintenance strategy.”
What are predictive maintenance tools?
In today’s fast-paced industrial landscape, the need for efficiency, reliability, and cost-effectiveness has never been more pronounced. Companies across various sectors are continually seeking ways to optimize their operations and minimize downtime. One of the most transformative approaches to achieving these goals is predictive maintenance. But what exactly are predictive maintenance tools, and how are they reshaping the future of asset management?
Predictive maintenance tools refer to a suite of technologies and methodologies designed to predict when equipment or machinery is likely to fail, thereby allowing for timely maintenance and repairs. Unlike traditional maintenance strategies, which are often reactive or scheduled at regular intervals, predictive maintenance leverages data and advanced analytics to anticipate issues before they become critical. This proactive approach not only improves operational efficiency but also extends the lifespan of assets and reduces overall maintenance costs.
At the heart of predictive maintenance is the integration of various technologies, including sensors, Internet of Things (IoT) devices, machine learning algorithms, and advanced data analytics. These tools work in tandem to monitor the condition of equipment in real-time, collecting vast amounts of data on parameters such as temperature, vibration, pressure, and more. By analyzing this data, predictive maintenance systems can identify patterns and anomalies that indicate potential failures.
One of the key components of predictive maintenance tools is the use of sensors and IoT devices. These devices are embedded in machinery and equipment, continuously gathering data on various operational parameters. For instance, vibration sensors can detect unusual oscillations in rotating machinery, while thermal sensors can monitor temperature fluctuations that might indicate overheating. The real-time data collected by these sensors is then transmitted to a centralized system for analysis.
Machine learning algorithms play a crucial role in predictive maintenance by processing and interpreting the data collected by sensors. These algorithms are trained on historical data, enabling them to recognize patterns and trends that are indicative of impending failures. Over time, the algorithms become increasingly accurate, allowing for more reliable predictions. For example, a machine learning model might identify that a certain combination of temperature and vibration levels typically precedes a motor failure, prompting maintenance personnel to take preventive action.
Data analytics is another vital aspect of predictive maintenance tools. Advanced analytics platforms can aggregate and analyze data from multiple sources, providing a comprehensive view of equipment health. These platforms often feature intuitive dashboards and visualization tools, making it easier for maintenance teams to interpret the data and make informed decisions. Additionally, predictive maintenance systems can generate automated alerts and notifications, ensuring that potential issues are addressed promptly.
The benefits of predictive maintenance tools extend beyond mere cost savings. By reducing unplanned downtime, companies can maintain higher levels of productivity and ensure the smooth operation of critical processes. This is particularly important in industries such as manufacturing, energy, and transportation, where equipment failures can have significant financial and operational repercussions. Furthermore, predictive maintenance can enhance safety by identifying and mitigating risks before they escalate into hazardous situations.
In the manufacturing sector, for example, predictive maintenance tools can monitor the condition of production line machinery, identifying wear and tear that could lead to breakdowns. By addressing these issues proactively, manufacturers can avoid costly disruptions and maintain consistent output. Similarly, in the energy industry, predictive maintenance can optimize the performance of power plants and distribution networks, ensuring a reliable supply of electricity.
Another significant advantage of predictive maintenance tools is their ability to extend the lifespan of assets. Regular maintenance, informed by accurate predictions, can prevent excessive wear and tear, thereby prolonging the useful life of equipment. This not only reduces the need for frequent replacements but also contributes to sustainability efforts by minimizing waste.
The implementation of predictive maintenance tools also fosters a culture of continuous improvement within organizations. By leveraging data-driven insights, companies can refine their maintenance strategies and operational processes, leading to ongoing enhancements in efficiency and performance. This iterative approach ensures that businesses remain agile and responsive to changing conditions and emerging challenges.
Moreover, predictive maintenance tools can facilitate better resource allocation. By identifying which assets require attention and when, companies can optimize their maintenance schedules and allocate resources more effectively. This targeted approach reduces the burden on maintenance teams and ensures that critical issues are prioritized.
While the advantages of predictive maintenance tools are clear, their successful implementation requires careful planning and execution. Organizations must invest in the necessary infrastructure, including sensors, IoT devices, and data analytics platforms. Additionally, they must develop the expertise needed to interpret and act on the insights generated by predictive maintenance systems. This often involves training maintenance personnel and fostering collaboration between different departments, such as operations, IT, and engineering.
In conclusion, predictive maintenance tools represent a paradigm shift in asset management and operational efficiency. By harnessing the power of data and advanced analytics, these tools enable companies to anticipate and address equipment issues before they lead to costly failures. The result is a more reliable, efficient, and sustainable approach to maintenance that benefits businesses across a wide range of industries. As technology continues to evolve, the capabilities of predictive maintenance tools will only grow, further cementing their role as a cornerstone of modern industrial practices.
The Future of Predictive Maintenance: Emerging Trends and Innovations
As predictive maintenance tools continue to revolutionize asset management and operational efficiency, the future holds even more promising advancements and innovations. The convergence of cutting-edge technologies and evolving industry practices is set to further enhance the capabilities of predictive maintenance, making it an indispensable component of modern industrial operations. Let’s delve into some of the emerging trends and innovations that are shaping the future of predictive maintenance.
Integration of Artificial Intelligence and Deep Learning
While machine learning has already made significant contributions to predictive maintenance, the integration of artificial intelligence (AI) and deep learning is poised to take these tools to the next level. AI algorithms can analyze vast datasets with unprecedented speed and accuracy, uncovering complex patterns and correlations that might be missed by traditional methods. Deep learning, a subset of AI, involves neural networks that can process unstructured data such as images and sound. This capability can be particularly useful for identifying visual signs of wear and tear or analyzing acoustic signals from machinery, providing a more comprehensive understanding of equipment health.
Edge Computing for Real-Time Decision Making
The rise of edge computing is another game-changer in the realm of predictive maintenance. Edge computing involves processing data closer to the source of generation, such as sensors and IoT devices, rather than relying solely on centralized cloud servers. This approach reduces latency and enables real-time decision-making, which is crucial for preventing sudden equipment failures. With edge computing, predictive maintenance systems can quickly analyze data on-site, generate immediate alerts, and even initiate automated corrective actions, thereby minimizing downtime and enhancing operational efficiency.
Digital Twins: A Virtual Mirror of Physical Assets
The concept of digital twins is gaining traction as a powerful tool for predictive maintenance. A digital twin is a virtual replica of a physical asset, created using real-time data and advanced simulation models. By mirroring the behavior and condition of the actual equipment, digital twins allow for detailed analysis and testing in a virtual environment. This enables maintenance teams to predict potential issues with greater accuracy and experiment with different maintenance strategies without disrupting real-world operations. Digital twins also facilitate remote monitoring and diagnostics, making it easier to manage assets spread across multiple locations.
Enhanced Human-Machine Collaboration
As predictive maintenance tools become more sophisticated, the role of human operators is evolving. Rather than replacing human expertise, these tools are enhancing human-machine collaboration. Maintenance personnel can leverage advanced analytics and AI-driven insights to make more informed decisions and perform their tasks more efficiently. Augmented reality (AR) and virtual reality (VR) technologies are also being integrated into predictive maintenance solutions, providing immersive training experiences and real-time guidance during maintenance activities. This synergy between humans and machines leads to improved accuracy, faster response times, and a safer working environment.
Predictive Maintenance as a Service (PMaaS)
The growing adoption of predictive maintenance is giving rise to new business models, such as Predictive Maintenance as a Service (PMaaS). PMaaS providers offer end-to-end predictive maintenance solutions, including sensors, data analytics, and monitoring services, on a subscription basis. This model allows companies to access advanced predictive maintenance capabilities without the need for significant upfront investments in infrastructure and technology. PMaaS also enables continuous updates and improvements to the predictive maintenance system, ensuring that businesses always have access to the latest innovations and best practices.
Sustainability and Environmental Impact
Predictive maintenance tools are not only transforming industrial operations but also contributing to sustainability efforts. By optimizing maintenance activities and extending the lifespan of assets, these tools reduce the consumption of raw materials and energy. Additionally, predictive maintenance can help identify and mitigate environmental risks, such as leaks or emissions, before they cause significant harm. As industries increasingly prioritize sustainability, predictive maintenance will play a crucial role in supporting environmentally responsible practices and achieving sustainability goals.
Regulatory Compliance and Standardization
As predictive maintenance becomes more widespread, regulatory bodies and industry organizations are developing standards and guidelines to ensure its effective implementation. These standards cover aspects such as data security, interoperability, and performance metrics, providing a framework for consistent and reliable predictive maintenance practices. Compliance with these standards not only enhances the credibility and reliability of predictive maintenance tools but also facilitates their integration into existing regulatory frameworks. This trend towards standardization is expected to drive broader adoption and trust in predictive maintenance solutions.
Conclusion: Embracing the Future of Predictive Maintenance
The future of predictive maintenance is brimming with possibilities, driven by advancements in AI, edge computing, digital twins, and more. These innovations are set to further enhance the accuracy, efficiency, and reliability of predictive maintenance tools, making them an integral part of modern industrial operations. As companies continue to embrace these technologies, they will unlock new levels of operational excellence, sustainability, and competitive advantage.
To fully realize the potential of predictive maintenance, organizations must stay abreast of emerging trends and invest in the necessary infrastructure and expertise. By fostering a culture of innovation and continuous improvement, businesses can harness the power of predictive maintenance to navigate the challenges of today’s industrial landscape and thrive in the future.
In summary, predictive maintenance tools are not just a trend but a transformative force that is reshaping the way industries manage their assets and operations. With ongoing advancements and a commitment to innovation, the future of predictive maintenance holds immense promise, paving the way for a more efficient, reliable, and sustainable industrial ecosystem.”
What does predictive maintenance involve?
In today’s fast-paced industrial landscape, the need for efficient and reliable operations has never been greater. Downtime, unexpected failures, and maintenance costs can significantly impact an organization’s bottom line. This is where predictive maintenance (PdM) comes into play. But what exactly does predictive maintenance involve? Let’s delve into the intricacies of this transformative approach.
Predictive Maintenance Defined
Predictive maintenance is a proactive strategy that uses data analysis tools and techniques to detect anomalies in your operation and potential defects in equipment and processes so you can fix them before they result in failure. Unlike traditional maintenance methods, which are either reactive (fixing something after it breaks) or preventive (fixing something at regular intervals regardless of its condition), predictive maintenance aims to predict when equipment failure might occur and to prevent it before it happens.
The Core Components of Predictive Maintenance
Predictive maintenance involves several key components, each playing a crucial role in ensuring the effectiveness of the strategy. These components include sensors, data collection, data analysis, and actionable insights.
Sensors and Data Collection
One of the foundational elements of predictive maintenance is the use of sensors to monitor the condition of equipment in real-time. These sensors can measure various parameters such as vibration, temperature, pressure, and humidity, among others. The data collected from these sensors provide a wealth of information about the operational status of the equipment.
Data Analysis
The next step involves analyzing the collected data to identify patterns and anomalies. Advanced data analytics techniques, including machine learning and artificial intelligence, are often employed to process and interpret the data. These technologies can predict potential failures by recognizing subtle patterns that may not be apparent to human operators. The analysis can be conducted in real-time, providing immediate insights into the health of the equipment.
Actionable Insights
The ultimate goal of predictive maintenance is to provide actionable insights that can be used to prevent equipment failures. This involves generating alerts and recommendations based on the data analysis. For instance, if the data indicates that a particular component is likely to fail within the next month, the system can alert the maintenance team to replace or repair the component before it causes a breakdown.
The Benefits of Predictive Maintenance
Understanding what predictive maintenance involves is only part of the equation. It’s also essential to recognize the myriad benefits it offers.
Reduced Downtime
One of the most significant advantages of predictive maintenance is the reduction in downtime. By predicting when equipment is likely to fail and addressing the issue beforehand, organizations can avoid unexpected breakdowns that can halt production and disrupt operations.
Cost Savings
Predictive maintenance can lead to substantial cost savings. By preventing unexpected failures, organizations can avoid the high costs associated with emergency repairs and replacements. Additionally, predictive maintenance allows for more efficient use of resources, as maintenance activities are only performed when necessary.
Extended Equipment Lifespan
Regularly monitoring and maintaining equipment based on its actual condition can extend its lifespan. Predictive maintenance helps identify and address minor issues before they escalate into major problems that can cause significant wear and tear on the equipment.
Improved Safety
Equipment failures can pose significant safety risks, especially in industries such as manufacturing, mining, and oil and gas. Predictive maintenance helps mitigate these risks by ensuring that equipment operates reliably and safely.
Enhanced Operational Efficiency
By minimizing downtime and optimizing maintenance activities, predictive maintenance can enhance overall operational efficiency. This allows organizations to maintain consistent production levels and meet their operational goals more effectively.
Implementing Predictive Maintenance
Implementing predictive maintenance involves several steps, starting with selecting the right technology and tools. Organizations need to invest in sensors and data collection systems that are compatible with their equipment. Additionally, they need to choose data analytics software that can process and interpret the collected data accurately.
Once the technology is in place, organizations need to establish a data management strategy. This involves collecting, storing, and analyzing data in a systematic and organized manner. It’s also essential to train the maintenance team on how to use the predictive maintenance tools and interpret the insights generated by the system.
Challenges and Considerations
While predictive maintenance offers numerous benefits, it’s not without its challenges. One of the primary challenges is the initial investment in technology and infrastructure. Implementing predictive maintenance requires a significant upfront investment in sensors, data analytics software, and other tools.
Another challenge is data management. Collecting and analyzing large volumes of data can be complex and time-consuming. Organizations need to have robust data management systems in place to handle the data effectively.
Additionally, predictive maintenance requires a cultural shift within the organization. Maintenance teams need to transition from a reactive or preventive mindset to a proactive approach. This may involve retraining staff and changing existing maintenance processes.
The Future of Predictive Maintenance
As technology continues to evolve, the future of predictive maintenance looks promising. Advances in artificial intelligence and machine learning are expected to enhance the accuracy and reliability of predictive maintenance systems. Additionally, the integration of the Internet of Things (IoT) is likely to play a significant role in the future of predictive maintenance, enabling more seamless and real-time data collection and analysis.
Predictive maintenance is revolutionizing the way organizations approach equipment maintenance. By leveraging data and advanced analytics, organizations can predict and prevent equipment failures, leading to reduced downtime, cost savings, extended equipment lifespan, improved safety, and enhanced operational efficiency. While there are challenges to implementing predictive maintenance, the benefits far outweigh the obstacles, making it a worthwhile investment for organizations looking to optimize their operations and stay competitive in today’s industrial landscape.
Predictive Maintenance: What It Involves and Why It Matters
In today’s fast-paced industrial landscape, the need for efficient and reliable operations has never been greater. Downtime, unexpected failures, and maintenance costs can significantly impact an organization’s bottom line. This is where predictive maintenance (PdM) comes into play. But what exactly does predictive maintenance involve? Let’s delve into the intricacies of this transformative approach.
Predictive Maintenance Defined
Predictive maintenance is a proactive strategy that uses data analysis tools and techniques to detect anomalies in your operation and potential defects in equipment and processes so you can fix them before they result in failure. Unlike traditional maintenance methods, which are either reactive (fixing something after it breaks) or preventive (fixing something at regular intervals regardless of its condition), predictive maintenance aims to predict when equipment failure might occur and to prevent it before it happens.
The Core Components of Predictive Maintenance
Predictive maintenance involves several key components, each playing a crucial role in ensuring the effectiveness of the strategy. These components include sensors, data collection, data analysis, and actionable insights.
Sensors and Data Collection
One of the foundational elements of predictive maintenance is the use of sensors to monitor the condition of equipment in real-time. These sensors can measure various parameters such as vibration, temperature, pressure, and humidity, among others. The data collected from these sensors provide a wealth of information about the operational status of the equipment.
Data Analysis
The next step involves analyzing the collected data to identify patterns and anomalies. Advanced data analytics techniques, including machine learning and artificial intelligence, are often employed to process and interpret the data. These technologies can predict potential failures by recognizing subtle patterns that may not be apparent to human operators. The analysis can be conducted in real-time, providing immediate insights into the health of the equipment.
Actionable Insights
The ultimate goal of predictive maintenance is to provide actionable insights that can be used to prevent equipment failures. This involves generating alerts and recommendations based on the data analysis. For instance, if the data indicates that a particular component is likely to fail within the next month, the system can alert the maintenance team to replace or repair the component before it causes a breakdown.
The Benefits of Predictive Maintenance
Understanding what predictive maintenance involves is only part of the equation. It’s also essential to recognize the myriad benefits it offers.
Reduced Downtime
One of the most significant advantages of predictive maintenance is the reduction in downtime. By predicting when equipment is likely to fail and addressing the issue beforehand, organizations can avoid unexpected breakdowns that can halt production and disrupt operations.
Cost Savings
Predictive maintenance can lead to substantial cost savings. By preventing unexpected failures, organizations can avoid the high costs associated with emergency repairs and replacements. Additionally, predictive maintenance allows for more efficient use of resources, as maintenance activities are only performed when necessary.
Extended Equipment Lifespan
Regularly monitoring and maintaining equipment based on its actual condition can extend its lifespan. Predictive maintenance helps identify and address minor issues before they escalate into major problems that can cause significant wear and tear on the equipment.
Improved Safety
Equipment failures can pose significant safety risks, especially in industries such as manufacturing, mining, and oil and gas. Predictive maintenance helps mitigate these risks by ensuring that equipment operates reliably and safely.
Enhanced Operational Efficiency
By minimizing downtime and optimizing maintenance activities, predictive maintenance can enhance overall operational efficiency. This allows organizations to maintain consistent production levels and meet their operational goals more effectively.
Implementing Predictive Maintenance
Implementing predictive maintenance involves several steps, starting with selecting the right technology and tools. Organizations need to invest in sensors and data collection systems that are compatible with their equipment. Additionally, they need to choose data analytics software that can process and interpret the collected data accurately.
Once the technology is in place, organizations need to establish a data management strategy. This involves collecting, storing, and analyzing data in a systematic and organized manner. It’s also essential to train the maintenance team on how to use the predictive maintenance tools and interpret the insights generated by the system.
Challenges and Considerations
While predictive maintenance offers numerous benefits, it’s not without its challenges. One of the primary challenges is the initial investment in technology and infrastructure. Implementing predictive maintenance requires a significant upfront investment in sensors, data analytics software, and other tools.
Another challenge is data management. Collecting and analyzing large volumes of data can be complex and time-consuming. Organizations need to have robust data management systems in place to handle the data effectively.
Additionally, predictive maintenance requires a cultural shift within the organization. Maintenance teams need to transition from a reactive or preventive mindset to a proactive approach. This may involve retraining staff and changing existing maintenance processes.
The Future of Predictive Maintenance
As technology continues to evolve, the future of predictive maintenance looks promising. Advances in artificial intelligence and machine learning are expected to enhance the accuracy and reliability of predictive maintenance systems. Additionally, the integration of the Internet of Things (IoT) is likely to play a significant role in the future of predictive maintenance, enabling more seamless and real-time data collection and analysis.
Moreover, the advent of digital twins—virtual replicas of physical assets—could revolutionize predictive maintenance. Digital twins allow for real-time monitoring and simulation of equipment performance, providing even deeper insights and more accurate predictions.
Conclusion
Predictive maintenance is revolutionizing the way organizations approach equipment maintenance. By leveraging data and advanced analytics, organizations can predict and prevent equipment failures, leading to reduced downtime, cost savings, extended equipment lifespan, improved safety, and enhanced operational efficiency. While there are challenges to implementing predictive maintenance, the benefits far outweigh the obstacles, making it a worthwhile investment for organizations looking to optimize their operations and stay competitive in today’s industrial landscape.
As we move forward, the continuous advancements in technology will only serve to make predictive maintenance more accessible and effective, solidifying its place as a cornerstone of modern industrial strategy. Organizations that embrace this proactive approach will be well-positioned to achieve greater operational resilience and long-term success.”