The automotive manufacturing landscape is undergoing a profound transformation. Assembly lines that once relied solely on human oversight and scheduled maintenance are now becoming intelligent, self-monitoring ecosystems. At the heart of this revolution is artificial intelligence—technology that’s redefining quality control, operational efficiency, and equipment reliability in automotive production.
Today’s leading manufacturers are implementing AI-powered visual inspection systems that can detect microscopic defects in milliseconds and predictive maintenance platforms that anticipate equipment failures before they occur. These innovations aren’t just incremental improvements—they represent a fundamental shift in how vehicles are built, promising higher quality, reduced costs, and unprecedented production efficiency.
The Rise of AI in Automotive Manufacturing
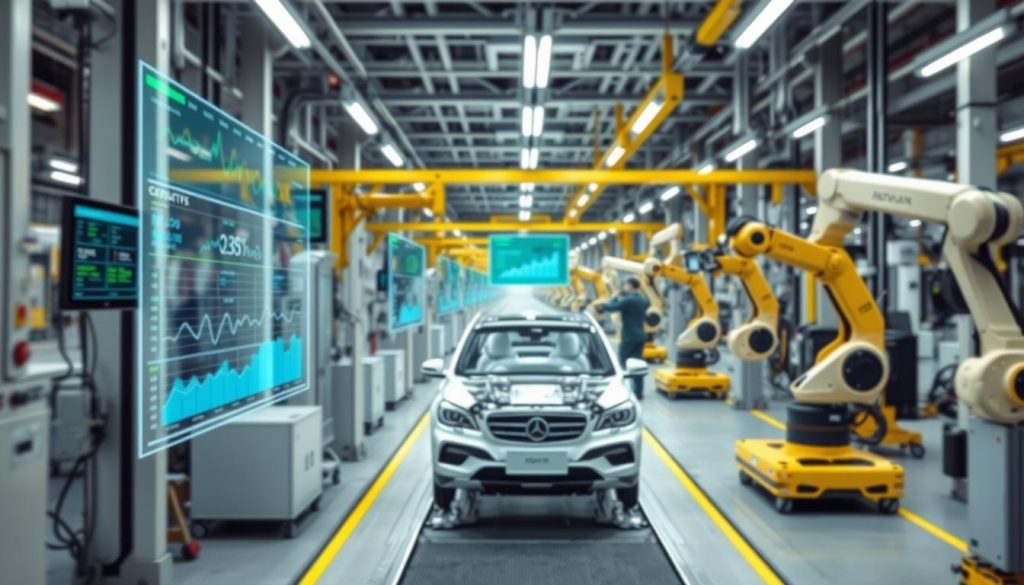
AI systems are revolutionizing automotive production lines with real-time monitoring and analysis
The automotive industry has always been at the forefront of manufacturing innovation, from Henry Ford’s assembly line to Toyota’s lean manufacturing principles. Today, we’re witnessing the next great leap forward as AI in automotive manufacturing transforms production facilities into smart factories.
According to recent industry data, nearly 44% of automotive manufacturers have already implemented some form of AI technology in their operations. This adoption is driven by compelling benefits: manufacturers report average productivity gains of 20%, quality improvements of 35%, and maintenance cost reductions of 25% after implementing AI solutions.
Two applications stand out as particularly transformative: AI-powered visual inspection and predictive maintenance. These technologies address critical pain points in automotive production—quality control and equipment reliability—while delivering measurable returns on investment.
AI-Powered Visual Inspection: Redefining Quality Control
Quality control has always been a critical concern in automotive manufacturing. Traditional inspection methods rely heavily on human inspectors who, despite their expertise, are subject to fatigue, inconsistency, and limitations in detecting certain types of defects. AI-powered visual inspection systems overcome these limitations, offering unprecedented accuracy, speed, and consistency.
AI visual inspection systems can detect microscopic defects invisible to the human eye
How AI Visual Inspection Works
At its core, AI visual inspection combines advanced camera systems with sophisticated machine learning algorithms. Here’s how the process typically unfolds:
Real-World Applications in Automotive Manufacturing
Paint Quality Inspection
One of the most widespread applications is in paint quality control. AI systems can detect issues invisible to the human eye, including:
- Microscopic bubbles and blisters
- Subtle color variations and mismatches
- Orange peel texture inconsistencies
- Dust particles and contamination
- Uneven application and drips
A major European automaker implemented an AI vision system for paint inspection and reduced defect rates by 38% while increasing inspection speed by 3x compared to manual methods.
Weld Quality Verification
Welding is critical for vehicle structural integrity and safety. AI inspection systems evaluate welds by analyzing:
- Weld bead geometry and consistency
- Porosity and inclusion detection
- Crack identification
- Fusion quality assessment
- Heat-affected zone analysis
A Japanese automotive supplier reported that their AI weld inspection system identified 23% more critical defects than traditional methods while reducing inspection time by 65%.
AI systems can analyze weld quality with precision that surpasses human inspection
Component Assembly Verification
Ensuring that all components are properly assembled is crucial for vehicle quality and safety. AI vision systems verify:
Business Impact of AI Visual Inspection
Benefits of AI Visual Inspection
- Detects defects invisible to human inspectors
- Operates 24/7 without fatigue or inconsistency
- Processes inspections in milliseconds
- Creates digital records for traceability and analysis
- Reduces warranty claims and recalls
- Improves customer satisfaction through higher quality
Implementation Challenges
- Requires significant initial investment
- Needs extensive training data for accuracy
- May require production line modifications
- Demands integration with existing systems
- Requires ongoing algorithm maintenance
- Necessitates workforce training and adaptation
ROI Case Study: Major Automotive Manufacturer
A leading North American automotive manufacturer implemented AI visual inspection across multiple production lines with impressive results:
Metric | Before AI Implementation | After AI Implementation | Improvement |
Defect Detection Rate | 82% | 98% | +16% |
Inspection Time Per Vehicle | 45 minutes | 12 minutes | -73% |
False Positive Rate | 14% | 3% | -79% |
Quality-Related Warranty Claims | 3.2% | 1.1% | -66% |
Annual Savings | – | $4.2 million | ROI in 14 months |
The manufacturer achieved complete ROI within 14 months and continues to see compounding benefits as the AI system learns and improves over time.
Predictive Maintenance AI: Preventing Downtime Before It Happens
AI predictive maintenance systems provide real-time insights into equipment health and performance
Unplanned downtime is the nemesis of manufacturing efficiency. In automotive production, a single hour of unexpected downtime can cost upwards of $1.3 million. Traditional maintenance approaches—either reactive (fix it when it breaks) or scheduled (maintain at regular intervals regardless of condition)—are increasingly inadequate in today’s high-pressure manufacturing environment.
AI-powered predictive maintenance represents a paradigm shift, enabling manufacturers to anticipate and address equipment issues before they cause production disruptions.
How AI Predictive Maintenance Works
IoT sensors collect real-time data that AI algorithms analyze to predict maintenance needs
Real-World Applications in Automotive Manufacturing
Robotic Systems Maintenance
AI monitors robotic welding, painting, and assembly systems to detect:
- Motor bearing wear patterns
- Calibration drift
- Hydraulic system pressure anomalies
- Control system irregularities
A German automotive plant reduced robot-related downtime by 37% after implementing AI predictive maintenance.
Press Line Optimization
Stamping press lines benefit from AI monitoring of:
- Hydraulic system performance
- Die wear patterns
- Lubrication system effectiveness
- Vibration signatures indicating misalignment
A U.S. manufacturer extended die life by 28% and reduced unplanned press downtime by 41% using AI predictive analytics.
HVAC System Efficiency
Factory climate control systems are monitored for:
- Compressor performance degradation
- Filter clogging patterns
- Refrigerant pressure anomalies
- Energy consumption deviations
A Japanese automotive facility reduced HVAC energy consumption by 23% while improving system reliability by 31% with AI-driven maintenance.
Conveyor and Transport System Monitoring
The complex conveyor systems that move components through automotive assembly plants are critical to production flow. AI predictive maintenance monitors:
AI systems continuously monitor conveyor performance to prevent costly production line stoppages
A major European automotive manufacturer implemented AI monitoring across their main assembly line conveyor system and reduced unplanned conveyor stoppages by 62%, saving an estimated €3.4 million annually in prevented downtime.
Business Impact of AI Predictive Maintenance
Key Benefits of AI Predictive Maintenance
Operational Benefits
Financial Benefits
Data from multiple automotive manufacturers shows significant improvements after implementing AI predictive maintenance
Case Study: Luxury Automaker’s Transmission Assembly Line
A premium European automotive manufacturer implemented AI predictive maintenance across their transmission assembly operations with remarkable results:
The manufacturer installed over 1,200 IoT sensors across critical equipment, collecting data on vibration, temperature, acoustics, power consumption, and other parameters. Their AI system analyzed this data in real-time, identifying patterns that preceded previous failures and alerting maintenance teams to developing issues.
Within 18 months of implementation, they achieved:
- 47% reduction in unplanned downtime
- 32% decrease in maintenance costs
- 28% improvement in mean time between failures
- 21% reduction in spare parts inventory
- ROI achieved in 11 months
Traditional Methods vs. AI-Driven Approaches
AI-powered inspection offers significant advantages over traditional manual methods
Visual Inspection Comparison
Aspect | Traditional Manual Inspection | AI-Powered Visual Inspection |
Inspection Speed | Minutes per component | Milliseconds per component |
Consistency | Variable (affected by fatigue, shift changes) | Highly consistent 24/7 |
Defect Detection Rate | 70-85% | 95-99% |
Microscopic Defect Detection | Limited by human vision | Can detect micron-level defects |
Data Collection | Manual recording, limited analysis | Automatic digital records, comprehensive analytics |
Scalability | Requires additional trained personnel | Easily scaled across production lines |
Continuous Improvement | Slow, based on manual feedback | Rapid, data-driven learning |
Maintenance Approach Comparison
The evolution of maintenance approaches shows a clear progression toward AI-driven predictive methods
Aspect | Reactive Maintenance | Preventive Maintenance | AI Predictive Maintenance |
Approach | Fix after failure | Fixed schedule regardless of condition | Condition-based with failure prediction |
Downtime | High unplanned downtime | Moderate planned downtime | Minimal, optimally scheduled downtime |
Parts Usage | Emergency replacements, high inventory | Often premature replacement | Just-in-time replacement, optimized inventory |
Labor Efficiency | Crisis management, overtime | Scheduled but often unnecessary work | Optimized scheduling, focused interventions |
Equipment Lifespan | Shortened by catastrophic failures | Standard expected life | Extended through optimal maintenance |
Cost Efficiency | High emergency costs | Moderate but includes unnecessary maintenance | Optimized, highest ROI |
Implementing AI in Your Automotive Manufacturing Operations
Successful AI implementation requires collaboration between domain experts and data scientists
Implementing AI in automotive manufacturing requires a strategic approach that balances technological capabilities with practical operational considerations. Here’s a roadmap for successful implementation:
Implementation Roadmap
Begin with a thorough assessment of your current operations, identifying key pain points and opportunities for improvement. Set specific, measurable goals for your AI implementation, such as “Reduce quality defects by 30%” or “Decrease unplanned downtime by 40%.”
Ensure you have the necessary infrastructure to collect, store, and process the data required for AI systems. This may include installing sensors, upgrading networks, and implementing data management platforms.
Begin with focused pilot projects in areas with high potential ROI. This allows you to demonstrate value quickly while learning valuable implementation lessons before scaling.
Successful AI implementation requires collaboration between domain experts (manufacturing engineers, maintenance technicians) and technical specialists (data scientists, AI engineers).
Ensure your AI solutions integrate seamlessly with existing manufacturing execution systems (MES), enterprise resource planning (ERP), and other operational technologies.
Invest in training programs to ensure your team understands how to work with and benefit from AI systems. Address concerns about job displacement by emphasizing how AI augments human capabilities.
Continuously measure results against your established goals, and be prepared to refine your approach based on real-world performance data.
Implementation Tip: When starting with AI in automotive manufacturing, focus first on high-impact, data-rich processes where improvements will deliver significant ROI. Visual inspection of high-value components and predictive maintenance for critical equipment that causes bottlenecks when down are excellent starting points.
Common Implementation Challenges and Solutions
Future Trends in AI for Automotive Manufacturing
The future of automotive manufacturing will feature increasingly autonomous and intelligent production systems
The evolution of AI in automotive manufacturing is accelerating, with several emerging trends poised to further transform production capabilities:
Edge AI and Real-Time Processing
Edge computing brings AI processing closer to the data source, enabling real-time analysis and decision-making without relying on cloud connectivity. This trend is particularly important for time-sensitive applications like in-line quality control and immediate equipment shutdown in case of detected anomalies.
Next-generation edge AI systems will feature more powerful processors capable of running sophisticated machine learning models directly on the factory floor, with minimal latency. This will enable more responsive control systems and faster intervention when issues are detected.
Digital Twins and Simulation
Digital twin technology—creating virtual replicas of physical assets, processes, and systems—is becoming increasingly sophisticated through AI integration. These digital twins enable:
Digital twins powered by AI enable virtual testing and optimization of manufacturing processes
Generative AI for Manufacturing
Generative AI, which can create new content based on training data, is finding applications in automotive manufacturing:
Design Optimization
Generative AI can suggest design modifications that improve manufacturability while maintaining or enhancing performance. For example:
- Optimizing component designs for automated assembly
- Suggesting material substitutions that reduce costs
- Redesigning parts to minimize waste in production
- Creating tooling designs optimized for specific manufacturing processes
Process Simulation
Generative models can create synthetic data for training other AI systems, particularly valuable for rare failure scenarios:
- Simulating uncommon defect types for visual inspection training
- Generating synthetic sensor data representing equipment failures
- Creating virtual test scenarios for autonomous systems
- Modeling extreme operating conditions to test system resilience
Collaborative AI and Human-Machine Teaming
The future of automotive manufacturing will feature more sophisticated collaboration between AI systems and human workers:
Augmented reality interfaces will enable seamless collaboration between workers and AI systems
Autonomous Manufacturing Systems
The long-term vision for AI in automotive manufacturing points toward increasingly autonomous production systems that can:
While fully autonomous factories remain on the horizon, each advancement in AI capabilities brings the automotive industry closer to this vision of highly adaptive, self-optimizing production systems.
Conclusion: The Transformative Power of AI in Automotive Manufacturing
The integration of AI into automotive manufacturing represents one of the most significant technological shifts in the industry since the introduction of robotics. AI-powered visual inspection and predictive maintenance are not merely incremental improvements to existing processes—they fundamentally transform how vehicles are built, quality is assured, and production assets are managed.
The benefits are compelling: higher quality products, reduced costs, increased production efficiency, and enhanced competitiveness in a rapidly evolving market. Manufacturers who successfully implement these technologies gain not only operational advantages but also valuable data assets that drive continuous improvement and innovation.
As AI technologies continue to evolve, the gap between early adopters and laggards will widen. Forward-thinking manufacturers are already moving beyond basic implementations to explore more advanced applications like generative AI, digital twins, and autonomous manufacturing systems.
The journey toward AI-powered manufacturing is not without challenges, but the potential rewards—both immediate and long-term—make it an essential strategic priority for automotive manufacturers committed to excellence and innovation.